Among several molecular targets that were proposed for fighting coronaviruses in general and have been been proposed again in the case of severe acute respiratory syndrome coronavirus 2 (SARS-CoV-2) infections, is a chymotrypsin-like cysteine protease known as the 3C-like protease (3CLpro) or the main protease (Mpro) [1, 2]. The enzyme is involved in proteolytic processing of coronavirus polyproteins indispensable for forming the replicase-transcriptase complex. Inhibition of Mpro blocks the viral replication, which in turn gives an antiviral effect.
A dominant approach to blocking Mpro is by using covalent inhibitors. Several potent compounds of this type have been already reported for SARS-CoV-2 Mpro [3–5]. However, the covalent active compounds have their issues, e.g. a propensity to interact with off-targets causing side-effects and toxicity. Hence it is also important to develop non-covalent Mpro inhibitors.
In order to aid the efforts along this track, we decided to perform a virtual screening (VS) campaign towards the identification of potential SARS-CoV-2 Mpro non-covalent inhibitors. The results of this work are freely available to parties interested in COVID-19 drug discovery via the webpage: www.cov2mpro.com [6]. In the current contribution we describe our protocol and its validation, and comment on some of the findings.
Virtual screening is a computational (in silico) procedure, the aim of which is to identify probable binders (active compounds) for a given molecular target:
While it is unrealistic to expect VS to find ready-for-market drugs, the screening may provide good-quality starting points (hits) for further medicinal chemistry programmes. With the VS being only the initial step, the screened compounds should have some space for structural optimization (not too large size) and be readily acquirable. In light of this, the chosen screening pool comprised 2,658,170 million molecules of 300–400 Da molecular weight, moderate lipophilicity (logP between 1 and 3), and “in-stock” status found in the ZINC database [7]. Additionally, we removed molecules with reactive or otherwise problematic functional groups or substructures.
As to choose which computational technique to employ for our problem, we decided to perform receptor-based screening by molecular docking simulations to the 6LU7 crystal structure of SARS-CoV-2 Mpro [5]. An alternative choice of QSAR/pharmacophore approaches (ligand-based) for our task would not be easily realizable because only scarce empirical data on SARS-CoV-2 Mpro inhibitors were available. Three docking programs (freely-available to academic researchers) were considered: AutoDock 4.2.6 (AD) [8], AutoDock Vina 1.1.2 (Vina) [9], and DOCK 6.9 (DOCK) [10, 11].
In order to validate the usefulness of these programs for docking-based virtual screening towards the discovery of non-covalent binders of the SARS-CoV-2 main protease, we performed 2 retrospective tests on available data pertinent to the main proteases of other β-coronaviruses. As these proteins are highly similar in sequence and structure, the results of such trials might be expected to give some reasonable indication of the software performance in the case of SARS-CoV-2 Mpro. The tests were supposed to answer the following questions:
How well do the programs reproduce binding poses of non-covalent inhibitors of β-coronaviruses Mpro reported in a few available crystal structures?
How good are they at finding true non-covalent inhibitors of SARS-CoV-1 Mpro among structurally similar decoys?
For the first test, we considered 6 Mpro structures from 3 different β-coronaviruses: SARS-CoV-1 (96% sequence identity with SARS-CoV-1 Mpro, 2 structures [12, 13]), MERS-CoV (51% sequence identity, 1 structure [14]), and Bat-CoV HKU4 (50% sequence identity, 3 structures [15]). The crystallographic ligands were redocked to their native structures with all 3 programs. The success rate of correct pose prediction was 1/6, 3/6, and 4/6, for AD, Vina, and DOCK, respectively. Considering the closest homologue of our target, that is Mpro of SARS-CoV-1, for which there are 2 crystal structures, each program gave a correct pose in 1 of 2 cases while failing in another.
For the second test, 46 non-covalent inhibitors of SARS-CoV-1 Mpro were collected from the literature [12, 13] and seeded with decoys found by DecoyFinder [16] (in the ratio 1 : 75, giving in total about 3500 compounds). The idea behind such a procedure is to check whether a given program/protocol is able to discern ‘true’ ligands from ‘false’ ligands (decoys). The set was docked to 3V3M and 4MDS structures of SARS-CoV-1 Mpro using all 3 considered programs. The results of this test are given as enrichment factors (EF, Figure 1 A) and receiver operating characteristic (ROC) curves in Figure 1 B and Table I.
Figure 1
A – Formula for calculating the enrichment factor (EF) at a given percent of the ranking. B – Results of validation set docking for particular combinations of software and structure presented as EF plots and receiver operating characteristic (ROC) curve. TPR – true positive rate, FPR – false positive rate. A dashed line is the y = x line (performance of random classification). C – 2-phenylfuran scaffold as a core for Mpro inhibitors with the examples of known inhibitors and the predictions from the presented virtual screening
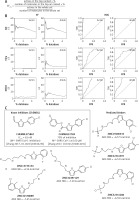
Table I
Results of validation set docking for particular combinations of software and structure
Variable | 3V3M structure | 4MDS structure | ||||||
---|---|---|---|---|---|---|---|---|
ROC AUC1 | EF1%2 | EF2%2 | EF5%2 | ROC AUC1 | EF1%2 | EF2%2 | EF5%2 | |
AutoDock 4 | 0.990 | 32.92 | 32.92 | 19.58 | 0.987 | 30.86 | 29.83 | 18.33 |
AutoDock Vina | 0.745 | 0.00 | 1.04 | 1.25 | 0.763 | 10.14 | 7.20 | 5.81 |
DOCK | 0.792 | 4.15 | 5.19 | 4.57 | 0.837 | 14.53 | 11.42 | 7.06 |
In this test, AutoDock 4 performed exceptionally well. In the cases of both structures, the program was able to pick the majority of ‘true’ ligands at the very beginning of the ranking list. The enrichment factors at the top 1% of database are here greater than 30, meaning that the program recognizes ‘true’ ligands with 30-times better efficacy than random choice would.
The performance of the 2 remaining programs was much worse. Vina did not find any ‘true’ ligand in the first 1% of the database (EF1% = 0) in the docking to 3V3M, and a moderate EF1% of 10.14 was found in the docking to 4MDS. DOCK performed better than that, but still with moderate success at best (3V3M EF1% = 4.15, 4MDS EF1% = 14.53). On the other hand, Vina and DOCK were significantly faster, which is an important factor if large libraries are to be screened. In the validation set, average docking times per molecule were: 207.7 s (AD), 24.5 s (Vina), and 27.7 s (DOCK).
Weighing up both the results of the validation tests and the speed, we decided to perform the proper screening task in a 2-step procedure. First, the whole set of over 2.6 million molecules was docked to SARS-CoV-2 Mpro (PDB: 6LU7, [5]) with DOCK. Then the best-scored 100,000 compounds were docked also with AutoDock 4 and AutoDock Vina. The ranking lists coming from these 2 stages are freely available via the webpage: www.cov2mpro.com. The page contains all the docked molecules in the native output format of each program, available for download in compressed archives. Furthermore, the on-site interface allows for access to the top scoring 10,000 compounds (by the AutoDock 4 scoring function). A user can filter the molecules according to several simple filters in order to download only a certain subset of the top scoring entries.
For a quantitative look-up of the interactions found in the top 10,000 entries, we performed a BINANA analysis [17] of the predicted binding modes. It revealed that the screened compounds are predicted to bind mostly in S1 and S2 subpockets of the active site, and a good number of them are able to touch S4 and S1’ sites. This suggests that the potential hits can be subject to further structural optimization for more dense interactions with subsites other than S1 and S2. Reaching a wider extent of active site surface is common to covalent inhibitors, and some of them span across the whole active site [5]. Further avenue for structural optimization may be an attempt to increase the number of hydrogen bonds that should give the ligands specificity. In the AD binding poses of the best-ranked 10,000 molecules, there are on average 2.7 ±1.5 hydrogen bonds.
With regard to chemical diversity, we conducted analysis of scaffolds and rings of the best-ranked 10,000 molecules. A query in the ChEMBL database [18, 19] revealed that only 1 of the scaffolds found in our predicted binders (2-phenylfuran) is present in the previously reported SARS-CoV-1 Mpro inhibitors. Furthermore, only a few scaffolds are substructures of inhibitors of other viral proteases. This means that our predicted binders, if confirmed to be hits, would provide chemical novelty to the group of Mpro inhibitors.
On the other hand, the fact that our VS procedure accidently ‘rediscovered’ the mentioned 2-phenylfuran scaffold as a core for Mpro inhibitors is gratifying (Figure 1 C). This scaffold (present in 6/10,000 top entries, rather in the lower middle part of the ranking, average AD estimate of binding free energy, FEB: –6.87 ±0.48 kcal/mol) is found in 2 relatively potent covalent inhibitors (Figure 1 C) reported previously [20]. This finding might be some remote indication of the correctness of the VS predictions.
In conclusion, the data obtained by our retrospectively validated VS protocol represent a large set of probable inhibitors of SARS-CoV-2 Mpro. The molecules are chemically diverse and novel compared to the known inhibitors. Nonetheless, they are readily acquirable from commercial suppliers. Moreover, there is a reasonable space for structural optimization both in terms of the properties of the molecules themselves, and the possibility of creating additional intramolecular contacts. Thus, the data form a good basis for pursuing an experimental medicinal chemistry programme aimed at the discovery of anti-SARS-CoV-2 drugs. Still, because of the in silico character of the screening results, it should be underscored that the predictions require experimental validation to see if the VS hits are true SARS-CoV-2 Mpro binders. If confirmed, the molecules might need to undergo structural optimization to achieve high activity and favourable physicochemical properties. Finally, pharmacological characterization will be needed to see if the putative confirmed hits are selective and safe.
Our VS results are provided freely via the webpage: www.cov2mpro.com. Hopefully, along with other recent in silico predictions [21–23], they will be useful to accelerate anti-SARS-CoV-2 drug discovery. The interested researchers are welcomed to use the data in their work. We are also open to co-operation proposals, in particular with respect to experimental testing of the VS predictions.