Introduction
Postoperative nausea and vomiting (PONV) is a highly relevant problem in anaesthesia care, being associated with patient discomfort and a significant economic impact such as prolonged length of stay in the post-anaesthesia care unit (PACU). Due to intensive research, prophylaxis and management of PONV were translated into comprehensive guidelines with high-grade evidence [1]. However, adherence to guidelines in perioperative care still seems to be rather poor [1–4]. Prophylaxis of PONV in everyday patient care is guided by well-known risk scores, though only a minority of patients receive an adequate PONV prophylaxis [5–9]. Translation of those clinical guidelines into standard operating procedures (SOP) and usage of an anaesthesia information management systems (AIMS) may aid clinicians in awareness of PONV risk and prevention, but their benefit is critically dependent on the details of implementation into practice [10–14].
In our tertiary referral hospital, anaesthetists are guided in PONV prophylaxis via a SOP that adopts current guideline recommendations and translates them into a feasible algorithm for patient care. When starting the AIMS for intraoperative data documentation, anaesthetists are offered a set of commonly used anaesthesia-specific medications for induction, antibiotic and PONV prophylaxis, which are tailored to the individual anaesthesia workplace. Anaesthetists may adopt these suggested medications, but are free to use and document any other medication they consider appropriate for the management of the patient.
In this study we retrospectively investigated the quality of PONV prophylaxis and therapy that is achieved with our current action bundle and analysed the potential requirement for change such as implementation of warning messages, aiming at best achievable perioperative guideline adherence.
Material and methods
Study design and population
The study was conducted retrospectively in compliance with the principles of the Declaration of Helsinki and the World Medical Association and after approval of the Ethics Committee of Hannover Medical School, Hannover, Germany (Chairperson Prof H.D. Tröger; approval number 1915–2013; 2013-Jul-24). Hannover Medical School is a tertiary referral hospital with approximately 30 000 anaesthesia procedures performed within the Department of Anaesthesiology and Intensive Care each year. The study interval was set from March 2013 (i.e. the beginning of institution-wide digital anaesthesia documentation) until March 2014 at Hannover Medical School. We retrospectively included all digitally documented anaesthesia procedures that involved patients aged 18 or older and were followed up in one of our PACUs. Patients who were transferred to an intensive care unit postoperatively and patients undergoing intracranial, obstetrical or cardiothoracic surgery were excluded from the study, because the assigning surgical departments use different documentation systems for medical data.
Data collection
In 2010, ANDOKlive (DATAPEC GmbH, Pliezhausen, Germany) was launched as the AIMS within the Department of Anaesthesiology and Intensive Care Medicine at Hannover Medical School. Digital documentation with ANDOKlive comprises anaesthetic patient data from the premedication visit until discharge from the PACU.
The mandatory fields for the premedication visit data are patient identification data, dropdown lists/shortlists for urgency of surgery, planned type of anaesthesia, planned surgery, ASA classification, metabolic equivalent tasks and the El-Ganzouri airway risk index items [15]. Existence of cardiovascular diseases, known allergies or long-term medication are also mandatory data (yes/no) with voluntary subgroup data fields. At the end of the premedication visit, date, time and duration of the premedication visit have to be documented. Patient identity data, gender and age are imported from the hospital medical information system (MIS) and do not require input by the anaesthetist. History of PONV or motion sickness as well as smoking status are represented by non-mandatory data fields. The default value for “history of PONV or motion sickness” was “absent”, and the default value for “history of smoking” was “non-smoking”.
Data were anonymised during extraction from the MIS database with retraceability of data via a unique internal ANDOKlive patient number that is different from patient identity numbers in the MIS system. From the intraoperative data, the first documented vital signs after presence of the anaesthetist in charge were obtained as well as intraoperative medication including dosage and time of administration (referenced to the beginning and/or the end of anaesthesia), durations of anaesthesia induction, surgery and reversal of anaesthesia as well as airway and anaesthesia techniques. From the PACU data, duration of attendance, first measures of vitals signs as well as the applied medication including dosage and time of administration (referenced to the beginning of the PACU time) were taken.
Study endpoints and main outcome measures
The primary endpoint of postoperative nausea and/or vomiting was reached if a patient was administered antiemetic medication (dexamethasone, 5-HT3 serotonin receptor antagonists, droperidol or dimenhydrinate) while being monitored in the PACU. Adequacy of PONV prophylaxis was evaluated with respect to the individual Apfel score and SOP of the Department of Anaesthesiology and Intensive Care Medicine that adopted 2011 recommendations for PONV prophylaxis [1, 5]. The Apfel score was calculated automatically based on the input of the Apfel score items during the premedication visit. Management of general anaesthesia as total intravenous anaesthesia (TIVA) was counted as a substitute for one antiemetic drug. For patients with an Apfel score of 0 or 1, no definite antiemetic prophylaxis is advised within the SOP. In this subgroup of patients, total intravenous anaesthesia (TIVA) was not counted as a prophylactic approach (patients with or without TIVA were considered as treated adequately). Administration of a prophylactic antiemetic drug in patients with an Apfel score of 0 or 1 was counted as an overshooting prophylaxis. Patients with an Apfel score of 2 or more should have received “Apfel score minus one” prophylactic drugs (TIVA counting as one prophylactic drug). Dexamethasone (4 to 8 mg) or methylprednisolone (40 mg) was considered as “administered correctly” if given within 30 min after anaesthesia induction and before the beginning of surgery. 5-HT3 antagonists (granisetron 0.35 to 3 mg, ondansetron 4 mg), droperidol (0.625 to 1.25 mg) and dimenhydrinate (0.5 to 1.5 mg/kg) were considered as “administered correctly” if given 60 min before the end of surgery.
Statistical analysis
Descriptive data are presented as median and interquartile range (IQR). Data are compared with nonparametric testing using the Kruskal-Wallis H test for multiple groups and the Mann-Whitney U test for comparison of two groups. Univariate and multivariate logistic regression are used to analyse risk prediction of PONV and association of PONV prophylaxis management with the occurrence of PONV. Data are presented as odds ratios (OR) with their respective 95% confidence interval (CI).
Because the Apfel score items “history of PONV or motion sickness” and “history of smoking” were non-mandatory data fields with “absent” as the set default values, we performed a data plausibility analysis of the item “history of smoking”. For plausibility analysis of the data item “smoking history” we also adopted the concept of the “Fragility Index” published by Walsh et al. for randomized controlled trial results [16]. The Fragility Index is defined as the smallest number of patients that have to change the group in a dichotomous classification, until a formerly significant p-value (< 0.05) exceeds 0.05. The smaller the Fragility Index is, the more fragile is a reported significant result. Area under the receiver operating characteristic (ROC) curve (AUC) was calculated (including binomial exact 95% CI) and used to derive the optimal risk predictive cutoffs for single risk factors with the DeLong method as well as to compare areas under the ROC curve [17].
None of the included cases was excluded because of missing or invalid data. Statistical analyses were calculated with SPSS 20.0 (SPSS, Chicago, IL) and MedCalc 14.10.2 (MedCalc Software, Ostende, Belgium).
Results
Patient characteristics
Twelve thousand seventy-eight anaesthesia procedures were retrieved from the database in the initial query. One thousand four hundred seventy-four procedures with patients younger than 18 years were excluded from further analysis. This resulted in the final dataset of 10 604 anaesthesia procedures that were analysed within this study. Patients underwent a broad spectrum of surgery, including 8159 procedures assigned to general and visceral surgery (9%), gynaecological surgery (18%), ear, nose and throat surgery (19%), ophthalmological surgery (10%), oral and maxillofacial surgery (4%), trauma surgery (10%) and urology surgery (7%). Baseline characteristics of the patients are shown in Table I. Five hundred eighty-nine (6%) of all patients suffered from PONV in the PACU, and PONV occurred at a median time of 35 min (IQR = 53 min) after admission. PONV in the PACU was associated with a longer stay (median: 122 min (IQR = 100 min) vs. 65 min (IQR = 51 min); p < 0.001). At admission to the PACU, patients developing a PONV presented with a higher pain score (NRS, numeric rating scale with 0 no pain, and 10 maximal pain) with a median of 5 (IQR = 5) versus 2 (IQR = 5) for patients without PONV (p < 0.001). The cumulative dose of piritramide for treatment of postoperative pain in the PACU (median: 9 mg (IQR = 10 mg) vs. 8 mg (IQR = 6 mg); p < 0.001), the heart rate (median: 86 bpm (IQR = 24 bpm) vs. 80 bpm (IQR = 22 bpm); p < 0.001) and the mean arterial pressure (median: 99 mm Hg (IQR 21 mm Hg) vs. 95 mm Hg (IQR 21 mm Hg); p < 0.001) at PACU admission were significantly higher in patients developing PONV. However, these differences probably lack clinical relevance.
Table I
Baseline characteristics of patients
[i] BMI – body mass index, CAD – coronary artery disease, MI – myocardial infarction, CKD – chronic kidney disease, NIDDM – non-insulin-dependent diabetes mellitus, IDDM – insulin-dependent diabetes mellitus. p-value: Mann-Whitney U test for the criterion present versus not present. OR – odds ratio (OR with respective 95% confidence interval (CI)) for PONV, comparing the criterion present versus not present.
Completeness and plausibility of electronic health records
In 446 (4%) patients, BMI was indeterminable because of missing data for body weight (12 (< 1%) cases), height (192 (2%) cases) or both values (242 (2%) cases). Three cases showed implausible data for body height (over 250 cm), likely caused due to additional erroneous digits during data input (e.g. “1852” instead of “185” cm). A preoperative haemoglobin level was available in 6499 (61%) of the patients at premedication visit. There were no missing values for gender, age, or the medical history items.
Data analysis suggested that smoking (as a risk-predictive item of the Apfel score) was associated with a reduced risk of coronary artery disease, congestive heart failure, chronic kidney disease and diabetes (Figure 1). Additionally, smoking was associated with a reduced probability of missing body weight or height measurements. While a reasonable correlation coefficient was found for a history of diabetes (r = 0.55; p < 0.001), arterial hypertension (r = 0.74; p < 0.001) and chronic kidney disease (r = 0.48; p < 0.001) with the sum of the documented pre-existing diseases, smoking did not show a clinically relevant correlation coefficient (r = –0.084; p < 0.001). We calculated Fragility Indices for the risk association of smoking with cardiovascular diseases (Table II). The results showed that 10 to 228 cases attributed to this counterintuitive risk association for smoking, raising the question of underreporting of smoking within the premedication visit reports.
Table II
Fragility Index values for “smoking” as a risk factor for cardiovascular diseases
Parameter | Fragility Index n (%) |
---|---|
Art. hypertension | 228 (10) |
BMI > 30 kg/m2 | 41 (2) |
Coronary artery disease | 10 (1) |
Congestive heart failure | 23 (2) |
Chronic kidney disease | 31 (6) |
Diabetes | 21 (2) |
Figure 1
Data plausibility analysis. Body mass index (BMI), history of diabetes, chronic kidney disease (CKD) and smoking are plotted as risk factors for the anamnestic items listed on the left side. The boxes depict odds ratios (OR) with their respective 95% confidence interval, the y-axes below the subheadings cross the x-axis each at 1. Boxes right of the y-axis show an increased OR (> 1), boxes left of the y-axis show a decreased OR (< 1). Boxes crossing the y-axis imply an OR that is not significantly different from 1. A BMI > 30 kg/m2 and a history of diabetes or CKD were shown to be associated with increased incidences of cardiovascular diseases (CAD, CHF, CKD), as expected. In contrast, data analysis suggested that a history of smoking was associated with a decreased incidence of CAD, CHF or CKD, diabetes (grey box) and with a decreased probability of missing weight/height values. The pattern of associations between smoking and cardiovascular diseases was different from the other depicted cardiovascular risk factors (diabetes and CKD), suggesting underreporting of smoking at the premedication visit
CAD – coronary artery disease, CHF – congestive heart failure, CKD – chronic kidney disease, PONV – postoperative nausea or vomiting.
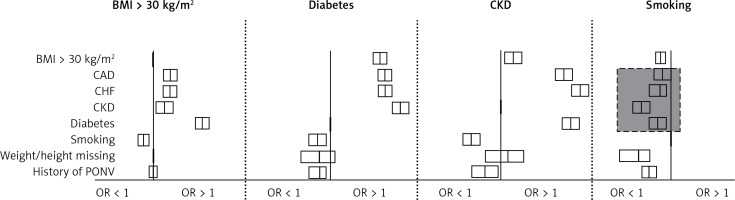
PONV – risk factor analysis
Female gender, non-smoking, a history of PONV or motion sickness and postoperative administration of opioids were each significantly associated with the occurrence of PONV in the PACU (Table I). PONV incidence was not significantly associated with the gender of the anaesthetist in charge of premedication (p = 0.507) or anaesthesia (p = 0.126). The probability of PONV increased linearly from 1% at Apfel score 0, to 15% at Apfel score 4. ROC analysis showed moderate accuracy for the Apfel score in risk prediction of PONV (AUC = 0.67; 95% CI: 0.66–0.68). Younger patient age as a continuous factor was associated with an increased incidence of PONV (Table I). The factor “younger age” with its best risk predictive cutoff at < 52 years was associated with an increased probability of PONV (OR = 1.6; 95% CI: 1.3–1.9), but did not significantly increase the AUC of the Apfel score (AUC = 0.68; 95% CI: 0.67–0.69; p = 0.30). PONV incidence was significantly different among the surgical departments (general and visceral surgery (8%), gynaecological surgery (6%), ear, nose and throat surgery (5%), ophthalmological surgery (3%), oral and maxillofacial surgery (4%), trauma surgery (9%) and urology surgery (3%); p < 0.001).
PONV – adherence to standard operation procedure (SOP)
Five thousand seven hundred forty-nine (54%) of the patients received a PONV prophylaxis that followed the recommendations of the SOP, while 2766 (26%) of the patients received an insufficient prophylaxis until the end of anaesthesia. Adherence to PONV prophylaxis varied among the surgical departments between 41% (gynaecological and ophthalmological surgery) and 73% (urology surgery). Comparing the different surgical departments, frequency of insufficient PONV prophylaxis correlated with mean PONV incidence (r = 0.805; p = 0.016). Insufficient PONV prophylaxis was associated with a PONV incidence of 11% versus 4% with correct prophylaxis (Figure 2). In comparison with correct prophylaxis, insufficient PONV prophylaxis represented a significant risk factor for the occurrence of PONV in the PACU (OR = 3.6; 95% CI: 3.0–4.3; p < 0.001). Two thousand eighty-nine (20%) of the patients received overshooting PONV prophylaxis, with 707 of these patients classified with an Apfel score of 0 or 1 (Figure 3). There was a significant trend to a higher incidence of insufficient prophylaxis with increasing Apfel score (p < 0.001) with 1585 patients with an Apfel score of 3 or 4 receiving insufficient prophylaxis.
Figure 2
Probability of PONV increased with insufficient prophylaxis. PONV prophylaxis was grouped into overshooting, correct or insufficient with respect to adherence to the PONV SOP (Kruskal-Wallis p < 0.001). While overshooting prophylaxis did not further reduce PONV probability compared to a correct prophylaxis (3.1% vs. 3.6%; p = 0.296), patients with insufficient prophylaxis showed a significantly increased PONV incidence compared to a correct prophylaxis (11.5% vs. 3.6%; p < 0.001)
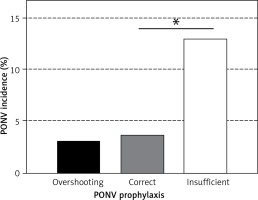
Figure 3
Probability of non-adherence to SOP differed with the Apfel score. Patients are grouped by their respective Apfel score. Whites bars represent patients with overshooting and grey bars with insufficient PONV prophylaxis. Patients with correct PONV prophylaxis are not shown. Patients with an Apfel score of 3 or more received insufficient PONV prophylaxis in more than 50% of cases (Kruskal-Wallis p < 0.001)
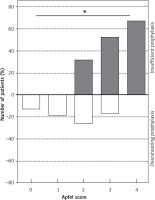
Analysis of decision triggers for insufficient PONV prophylaxis
Prevalence of pre-existing diseases as decision triggers for balanced versus total intravenous anaesthesia did not differ clinically relevantly between patients with insufficient and correct PONV prophylaxis (mean prevalence: arterial hypertension 35% versus 34% (p = 0.49), coronary artery disease 11% versus 14% (p = 0.005), chronic kidney disease 6% versus 5% (p = 0.46), heart failure 12% versus 12% (p = 0.71)). Two hundred thirty-three patients with insufficient PONV prophylaxis were known to suffer from diabetes (a relative contraindication for dexamethasone), while 2211 non-diabetic patients with insufficient PONV prophylaxis would have been potentially eligible for administration of dexamethasone. Within the subgroup of patients with a single missed prophylactic measure, 1846 (83%) patients would have been eligible for dexamethasone, 1437 (65%) for a TIVA, 2039 (92%) for a 5-HT3 antagonist and 2215 (99%) for droperidol (Figure 4).
Figure 4
Number of missing PONV prophylaxis measures. Relative frequency of the number of missed PONV prophylaxis measures is shown. The height of the bar for an individual medication/measure represents the relative relevance of the medication/measure in comparison to the others for achieving correct prophylaxis
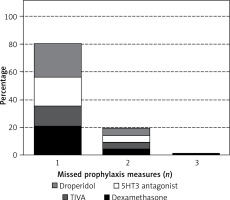
PONV – management in the PACU
Four hundred ninety-two (84%) patients with PONV needed a single medication for symptom release, and 97 (16%) were treated with up to four sequential antiemetic substances. Forty-five (9%) of the patients who suffered from PONV in the PACU received a drug that had already been administered prior to admission to the PACU, and thus according to the guidelines should not have been used for therapy again. Two thousand four hundred forty-nine (23%) of all patients were discharged from the PACU with an insufficient PONV prophylaxis.
Discussion
This study shows that adherence to PONV prophylaxis, despite the use of AIMS software and decision support for the suggested antiemetic drugs, was sufficient in only 54% of all anaesthesia procedures. Patients were discharged from the PACU with insufficient PONV prophylaxis in 23% of all cases. The current bundle of actions for PONV prophylaxis was associated with an overshooting antiemetic prophylaxis in patients with a low risk for PONV (Apfel score 0 or 1), and a trend to insufficient prophylaxis in patients at highest risk (Apfel Score 3 or 4) for PONV. More than 90% of the patients with insufficient antiemetic prophylaxis could have reached the aims of the SOP and current guidelines with administration of a 5-HT3 antagonist or droperidol at the end of surgery. The patients’ history of smoking habits was recorded as a voluntary data field during the premedication visit and analysis of these data suggested underreporting of smoking. If the history of smoking is included in PONV risk prediction, data acquisition has to be changed from voluntary to mandatory entry and without setting a default value.
Data quality of AIMS is generally thought to be higher than with paper-based documentation, but critically depends on the user, the type of data input and the configuration of the software [10, 18–22]. Translation of guidelines into local standard operating procedures and standardised digital algorithms may improve guideline adherence, but depends on the design and complexity of the algorithms and the validity of included patient data [12–14, 23].
The PONV incidence of 5% in our study was considerably lower than to be expected from previous studies [1, 14]. Because we did not record PONV after discharge from the PACU, the entire incidence in our study collective is likely higher than reported. However, compliance with PONV prophylaxis guidelines in our study was low, as already reported before [1].
Timing for individualised insufficient PONV prophylaxis warning messages might be placed better at the end of surgery than during induction of anaesthesia, because induction is known to be associated with reduced vigilance of anaesthetists for monitoring data [24]. We suggest re-evaluating adherence to correct PONV prophylaxis at the end of the PACU stay with an electronic reminder because of the relevant number of patients who were discharged with insufficient PONV prophylaxis and therefore were prone to develop nausea and vomiting on the peripheral ward [25]. While improvement of PONV guideline adherence is likely to be achieved with extended and automatic perioperative patient data analysis, external evidence regarding PONV prophylaxis always has to be weighed against consideration of the patients’ expectations and individual clinical findings [26]. At discharge from PACU, patients and caregivers can seek consent on whether the patient is reluctant or willing to receive several intravenous anti-emetics on the basis of individual PONV risk and personal preferences. An electronic reminder at discharge could trigger this personalised PONV prophylaxis approach.
This study was conducted in a retrospective manner and therefore is limited to associative relationships of the examined factors with PONV. PONV in itself was not explicitly documented by the staff in the PACU, although this data field existed for documentation. Therefore, PONV as the primary endpoint of the study has indirectly been defined by the need for antiemetic medication in the PACU. For a future prospective approach, the occurrence of PONV in itself has to be changed into a mandatory data field without a set default value. We only measured the incidence of PONV in the PACU. This underestimates the true incidence caused by PONV events in the peripheral ward. We accepted this limitation, because digital data acquisition ends with discharge from PACU and the feasibility of standardised digital recommendations for the improvement of PONV prophylaxis was the targeted aim of our study. We evaluated opioid therapy in the PACU for this study, but did not estimate opioid dosage and timing during anaesthesia, which also might be related to PONV occurrence due to altered demand of postoperative analgesics. History of smoking and past PONV or motion sickness were non-mandatory data fields within the premedication visit data acquisition. While there was some evidence for underreporting of smoking, data analysis for history of PONV or motion sickness was not feasible in the same manner. Because a positive history of smoking and PONV or motion sickness influenced PONV incidence in opposite directions, we consider it unlikely that Apfel scores were calculated with a systematic error. Nonetheless, future studies should change these two Apfel score items into mandatory data fields. Additional mandatory data fields for the premedication visit will increase data concordance, but also increase the duration of premedication visit documentation. Therefore, careful consideration and data analysis prior to the change of data fields from voluntary to mandatory are necessary.
In conclusion, PONV remains a frequent postoperative complication despite evidence-based guidelines. Even with the implementation of electronically assisted advice for PONV management, patients seem to be exposed to insufficient PONV prophylaxis. Though rarely seen, anti-emetic substances expose patients to potential adverse events, and both patients and doctors should seek consent on the risks and benefits of individual PONV prophylaxis beyond guideline evidence. If PONV risk factors are assessed using voluntary data fields during preoperative assessment, risk factors are likely to be underreported. Adherence to correct PONV prophylaxis should be re-evaluated systematically before discharge from the PACU, because many patients do not receive sufficient PONV prophylaxis during surgery or postoperative recovery.