Introduction
In affluent countries, atherosclerosis is the primary underlying cause of mortality and morbidity [1–3]. Coronary artery disease (CAD) accounts for 20% of all deaths in European Union countries and is the most common form of cardiovascular disease (CVD) [2, 3]. The available literature indicates that there is a lack of adequate public awareness regarding the relationship between CVD and atherosclerosis as the leading cause of mortality. Despite substantial medical, genetic, epidemiological, and preventive advancements in our understanding of atherogenesis during the past two decades, society’s awareness of cardiometabolic diseases is still practically unaltered [3].
Risk scores derived from classical risk factors such as hypertension, hypercholesterolemia, pre-diabetes, diabetes, and cigarette smoking allow us to predict adverse CVD events to a certain degree. However, there are limitations in the current risk scores, especially when assessing younger patients [4–6]. This is because traditional risk factors and civilization diseases, unless concentrated and severe, require time to cause detrimental health impacts [7]. For example, using age as a standalone factor, with a cut-off value of 55 years, can be nearly as accurate as the Framingham Risk Score (FRS) in predicting cardiac events. This is equivalent to screening every 5 years in Framingham, with a 10-year risk cutoff of 8% [7]. Also, the risk score charts that are currently available are not very useful for routine risk assessment for the primary prevention of CAD [5]. Furthermore, genetic determinants of disease significantly influence risk in younger patients [8]. However, there is ongoing disagreement as to whether this is simply due to a genetic predisposition to CAD or a heightened genetic susceptibility to traditional risk factors, such as an unhealthy lifestyle. Thus, there is a need for novel methods to identify people who are at risk of developing CAD, including those based on advancements in basic scientific research [2].
For a long time, there have been efforts to evaluate genetic risk by identifying gene variants strongly linked to atherosclerotic cardiovascular diseases (ASCVD) in gene-candidate investigations. Nevertheless, this method proved to be ineffective in forecasting the risk of CVD [9]. In 2007, Samani et al. published the first genome-wide association study (GWAS) on CAD. Since then, more than 150 gene loci related to CAD have been found [10]. Interestingly, most of these single nucleotide polymorphisms (SNPs) are found in genes not previously thought to be associated with atherosclerosis. Additionally, some are located in genes involved in lipid metabolism, insulin sensitivity, coagulation, inflammation, and vascular tone [11].
The polygenic risk score (PRS) is a weighted sum of the risk posed by multiple SNPs associated with the disease being analyzed [12]. To establish a PRS, the SNPs and their corresponding effect sizes, which quantify the association between the SNP and the disease, must be determined using an external GWAS-derived dataset. Next, we can verify the extensive correlation between SNPs across the genome using linkage disequilibrium [12]. Utilizing PRS based on these genetic variations has enhanced the accuracy of predicting cardiovascular events [13].
Statins are the primary therapy for lipid disorders and the most effective tool for preventing CAD in primary and secondary prevention [14]. In both primary and secondary prevention, the number needed to treat (NNT) for statins required to prevent one cardiovascular death is similar, between 15 and 30, and is comparable across different age groups [15]. Furthermore, the available data show that PRS can effectively predict the potential advantages of lipid-lowering therapy (LLT) [16]. Indeed, people with high genetic risk have a higher risk of subclinical atherosclerosis and benefit significantly more from statin treatment to prevent the first CVD event [2, 17, 18]. PRS may enable optimization of the NTT related to the use of statins for primary and secondary prevention of cardiovascular events [16, 17]. One recent study found that a PRS score derived from 27 SNPs accurately predicted adverse cardiovascular events and the magnitude of a response to treatment with a proprotein convertase subtilisin/kexin type 9 (PCSK-9) inhibitor (evolocumab), compared with a score derived from 6 million SNPs in people of European ancestry [18].
One important aspect to consider regarding the relevance of this 27-SNP score to the Polish population is that the above experiment included patients of European descent, and patients recruited from over 60 sites in Poland, Czechia, Slovakia, and Ukraine [19]. Improved identification of at-risk individuals, particularly among the youth, continues to be a critical public health concern. Early detection (the earlier, the better) of individuals who are at risk will facilitate prompt intervention, including education, lifestyle modifications, and LLT, all aimed at mitigating the mortality risk associated with cardiometabolic disorders [20].
It is worth noting that clinical risk estimators that are widely employed in Europe were initially developed for historical cohort studies involving middle-aged adults. As a result, their performance is comparatively inferior when applied to younger populations or individuals with non-European ancestry [21].
Hence, this study’s main aim is to create a new PRS for the Polish population and assess its utility in predicting mortality during 8 years of follow-up in the nationwide LIPIDOGEN2015 population. The secondary study goal is to assess the association between the lipid profile, statin/LLT treatment, and outcomes stratified by PRS score.
Material and methods
Subjects
Clinical and anthropometric data for each patient will be obtained from the nationwide cross-sectional study LIPIDOGRAM and LIPIDOGEN2015, conducted in 2015–2016 [22, 23]. In this program, a group of 438 physicians (investigators) working in 398 public or private primary health care ambulatory clinics in 16 central administrative regions of Poland (voivodeships) were selected (Figure 1).
Figure 1
Study design and methodology aimed at determining the risk of adverse cardiovascular events based on the analysis of common genetic variants in an 8-year follow-up of the LIPIDOGEN2015 population using the polygenic risk score (PRS)
DNA – deoxyribonucleic acid, HDL-C – high-density lipoprotein cholesterol, LDL-C – low-density lipoprotein cholesterol, SNPs – singe nucleotide polymorphisms, TC – total cholesterol, TG – triglycerides.
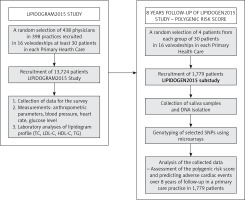
The details of the LIPIDOGRAM and LIPIDOGEN2015 study have been described elsewhere [22]. Briefly, all consecutive individuals > 18 years old, who were under the care of a physician-investigator in a primary care clinic, voluntarily seeking medical assistance for any medical reason, were included. The exclusion criteria were diagnosed dementia or mental illness, which could lead to the inability to give informed consent or the patient’s refusal to consent to participate in the study [23]. The LIPIDOGEN2015 project collected information for each participant using personal questionnaires regarding chronic diseases and their treatment, lifestyle (diet, physical activity, smoking), CVD family history and demographic data such as age, gender, place of residence and level of education. All patients underwent anthropometric measurements (height, body weight, waist circumference, and hip circumference), blood pressure, heart rate, fasting glucose, and lipid profile samples that were taken on the day of patient’s inclusion in the study. Study characteristics of patients participating in the LIPIDOGEN2015 study are presented in Table I.
Table I
Clinical characteristics of the LIPIDOGEN2015 study sample (N = 1779)
Additionally, 2 ml of saliva was collected from each patient for DNA isolation and preservation for genetic analyses. Follow-up data on hospitalization and cardiovascular and cause-specific mortality, as well as all-cause mortality, will be collected in the given project to verify a minimum follow-up period of 8 years for every patient (Figure 1).
Genetic analyses
DNA samples were quantified using Quant-iT dsDNA Broad Range Assay Kits (Invitrogen, Carlsbad, CA, USA) and NanoDrop 2000 (Thermo Fisher Scientific Inc., Waltham, MA, USA) to determine DNA quality and concentration. The DNA samples’ concentration was normalized to 50 ng/µl. DNA samples then underwent PCR reaction for sex verification [24]. All the DNA samples were genotyped using Infinium Global Screening Array-24+ v3.0 Kit microarrays (Illumina, San Diego, CA, USA), according to the protocol provided by the manufacturer. Briefly, DNA samples were amplified, then enzymatically fragmented and hybridized to the BeadChips. Afterwards, the BeadChips underwent extension and X-staining processes. Next the BeadChips were scanned using iScan (Illumina Inc., San Diego, CA, USA). Microarrays’ raw fluorescence intensity data were then converted to genotypes using Genome Studio 2.0. Standard microarray quality control protocols, such as filtering on sample call rates (> 0.94) or SNP genotyping rates (10% GenCall > 0.4) were executed. The results were exported from GenomeStudio using PLINK Input Report Plug-in v2.1.4 by forward strand. Genetic data were filtered; thus only variants with minor allele frequencies above 5% were included. Subsequently, Hardy-Weinberg equilibrium was tested and SNPs with a p-value > 0.05 were excluded from the association analysis. Information on the selected SNPs is presented in Table II.
Table II
List of polymorphisms that will serve to calculate a polygenic risk score
[i] PCSK9 – proprotein convertase subtilisin/kexin 9, PLPP3 – phospholipid phosphatase 3, CELSR2 – cadherin EGF LAG seven-pass G-type receptor 2, 2MIA3 – melanoma inhibitory activity member 3, WDR12 – WD repeat domain 12, MRAS – muscle RAS oncogene homolog, PHACTR1 – phosphatase and actin regulator 1, ANKS1A – ankyrin repeat and SAM domain-containing protein 1A, TCF21 – transcription factor 21, LPA – lipoprotein(A), ZC3HC1 – zinc finger C3HC-type containing 1, CDKN2B-AS1 – cyclin dependent kinase inhibitor 2B antisense RNA 1, ABO – alpha 1-3-N-acetylgalactosaminyltransferase and alpha 1-3-galactosyltransferase, CXCL12 – C-X-C motif chemokine ligand 12, CNNM2 – cyclin and CBS domain divalent metal cation transport mediator 2, ZPR1 – ZPR1 zinc finger, SH2B3 – SH2B adaptor protein 3, HNF1A – hepatocyte nuclear factor 1 homeobox A, COL4A1 – collagen type IV alpha 1 chain, HHIPL1 – hedgehog interacting protein-like 1, ADAMTS7 – ADAM metallopeptidase with thrombospondin type 1 motif 7, SMG6 – SMG6 nonsense mediated mRNA decay factor, RASD1 – Ras related dexamethasone induced 1, UBE2Z – ubiquitin conjugating enzyme E2Z, SMARCA4 – SWI/SNF related, matrix associated, actin dependent regulator of chromatin, subfamily A, member 4, KCNE2 – potassium voltage-gated channel subfamily E regulatory subunit 2.
Statistical analysis
Patients will be stratified by their genetic risk score into quintiles. Similarly to other studies on this issue, the lowest genetic risk will be defined as quintile 1, the highest as quantile 5. Quintiles 2-4 will be described as intermediate genetic risk [17, 18]. Continuous variables will be compared using the Wilcoxon rank sum test. Additionally, the Jonckheere-Terpstra test for a trend and Cochrane-Armitage or similar tests for trends will be used to evaluate changes in continuous variables and proportions across groups stratified by genetic risk score. If the assumptions of hazard proportionality are confirmed, Cox proportional hazard regression will be carried out to assess the association between quantiles of PRS, as well as standardized PRS and major adverse events defined as death, myocardial infarction, and stroke. The analysis will be adjusted for clinical variables. A p-value of < 0.05 will be considered statistically significant. A sample of 1779 persons is sufficient to detect a significant (at α = 0.05) association between the 27-SNP PRS and the occurrence of CAD with a power of 81.9%. Calculations were performed under conservative settings – the prevalence of CAD was assumed to be 5%, and the proportion of variance explained by genetic effects was assumed to be 0.011.
Results
Commonly occurring genetic variants listed in Table II will be correlated with outcomes. We intend to develop a preventive model based on the detailed clinical variables of patients from the LIPIDOGEN2015 study and the outcomes of SNP genotyping analyses utilizing DNA microarrays. The PRS created for the Polish population will estimate an individual’s genetic susceptibility to a specific trait or disease. We will select as features the conventional risk factors for CAD already included in several widely used risk scales, for which data are available. We will include additional non-genetic attributes such as diet, medications, and lifestyle factors to improve the model’s performance. Using a logistic regression framework, we will generate different risk prediction models, each with a different subset of features.
Discussion
ASCVD is classified as the main cause of loss of life years (LLY) due to disability or premature death among adults [1, 25]. GWAS studies do not fully elucidate the causal mechanisms of CVD. Further stratification of the genetic risk of developing CVD events will enable easier access to health care for patients with increased risk of cardiometabolic diseases and their complications [26]. This will enable initiation of early preventive measures to avoid loss of full-fledged functioning in everyday life and to prevent premature death due to CVD events [26]. The extended latency period of CAD allows for early implementation of preventive measures; however, it also necessitates the identification of patients at CVD risk during the asymptomatic phase [26]. Risk scales that are derived from traditional risk factors, including Framingham [27], SCORE [28], PROCAM [29], Reynolds [30], the more recent SCORE-2 and SCORE-OP, which were included in the European Society of Cardiology (ESC) Prevention guidelines 2021 [31], and the most recent SCORE2-Diabetes [32], are to some degree effective in identifying patients who are at CVD risk. The Pol-SCORE scale was developed for the Polish population in 2015 and was used to assess the risk of death from cardiovascular causes within 10 years, including very elderly patients (> 65 years of age) [33].
Nevertheless, the utility of these scores is significantly diminished when applied to patients younger than 55 [31]. Most frequently administered in the United States to patients younger than 40, the Framingham Risk Score as a tool for identifying high-risk individuals is ineffective [34]. The SCORE risk scale (as well as SCORE2), widely utilized in Europe, is also limited to patients 40 or older. Furthermore, it has deficiencies in identifying high-risk patients in the youngest age categories [35]. As previously stated, this phenomenon can likely be attributed to the gradual accumulation of detrimental effects caused by diabetes, hypercholesterolemia (excluding familial hypercholesterolemia [FH]), obesity, and unhealthy behaviors such as smoking [23, 36, 37].
The risk of premature CAD (defined as age 55 or younger in men and 60/65 or younger in women) is influenced more by genetics than by environmental factors [38]. The prevalence of CAD among patients under 60 is 5.9% for women and 6.3% for men, although more recently, especially in the post-COVID19 period, it has increased to as high as 10–15% in some countries [25, 39]. In the case of premature CAD, the subclinical atherosclerosis process likely begins 10–20 years earlier. Additionally, there is a positive correlation between age and the pathophysiology of atherosclerosis [40].
A method for accumulating data on genetic variants associated with specific disease traits is known as PRS [38, 41]. GWAS establish the association between gene variants and specific diseases. PRS may capture an increased genetic risk of a particular disease, based on numerous frequently occurring variants, in accordance with the principle “common disease – common variant” [38], as GWAS methodology typically identifies only variants that occur with a frequency greater than 5%. More than 150 gene loci have been identified as being associated with CAD [41]. These loci have achieved an accepted significance level of less than 5 × 10–8 in studies that have tested millions of gene variants multiple times [41].
Therefore, if the PRS for a particular disease trait is validated for the population, it may be possible to reliably locate each individual’s risk on the genetic risk scale of developing a certain disease or its complications. Prior PRS associated with CAD were determined by aggregating the number of disease-related variants, irrespective of the degree of association with the disease trait underlying the condition [42]. At this time, variants are weighted according to the severity of their association with the disease trait as a result of data collection, and several methods exist for accomplishing this [43]. Also, the establishment of population biobanks containing accessible clinical data makes this much more feasible.
As a result, PRS is currently more dependable than when the concept of genetic risk estimation was first conceived. Theoretically, PRS could identify patients at risk for CAD earlier in life, even as early as after birth; this would significantly alter the approach to primary (primordial) prevention of atherosclerotic diseases [44]. Assuming a liability threshold model, in which CAD manifests itself upon reaching a particular risk threshold in a continuous polygenic score distribution, risk prediction is frequently modelled using the PRS. This continuous measure is normally distributed in the population. However, the PRS score may be a risk indicator, but is not a diagnostic test for CAD. It should be evaluated like other continuously monitored conventional risk factors, including systolic blood pressure and LDL cholesterol [45]. For example, the PRS model for CAD constructed by Busby et al. included 29,389 individuals from various cohorts and genetic origin groups. This model classified 12–24% of individuals as having high genetic risk. Reclassifying borderline or intermediate 10-year risk of ASCVD using this risk factor improved the assessment of both CAD and ASCVD (net reclassification improvement [NRI] = 13.14% [95% CI: 9.23–17.06%] and NRI = 10.70% [95% CI: 7.35–14.05], respectively) in 9691 individuals [46]. According to these analyses, utilizing genetic information in PRS as risk enhancers improves ASCVD risk assessment, defining a strategy for managing ASCVD prevention [47]. Isgut et al. conducted research to validate the clinical utility of incorporating four PRS derived from SNPs (194, 46K, 1.5M, and 6M) in conjunction with conventional risk factors for predicting myocardial infarction (MI), ischemic heart disease (IHD), and premature MI occurrence before the age of 50 years [48]. They confirmed that clinical and lifestyle factors, not independent of genetic susceptibility, contribute to the increased risk of CVD in middle-aged individuals, and indicated that polygenic risk assessment may be useful for predicting risk at birth when other risk factors are unknown, thereby identifying individuals most susceptible to developing heart disease [48].
Over the past 5 years, extensive data on PRS and the incidence of CVD have been published. The PRS employed in those investigations ranged from 27 to more than 6 million SNPs. Based on data from primary prevention trials such as JUPITER (Justification for the Use of Statin in Prevention: An Intervention Trial Evaluating Rosuvastatin), ASCOT (Anglo-Scandinavian Cardiac Outcomes Trial), and the secondary prevention trials CARE (Cholesterol and Recurrent Events) and PROVE-IT TIMI 22 (Pravastatin or Atorvastatin Evaluation and Infection Therapy–Thrombolysis in Myocardial Infarction 22), Mega et al. investigated the PRS score based on 27 SNPs [17]. The authors demonstrated the potential utility of this score in predicting initial and recurrent cardiovascular events and its potential function in predicting response to statin therapy [17]. In the post-hoc analysis of the FOURIER (Further Cardiovascular Outcomes Research with PCSK9 Inhibition in Subjects With Elevated Risk), a 27-SNP risk score was equivalent to a 6 million SNP risk score in terms of the incidence of severe vascular and coronary events [18]. The 27-SNP score and the 6 million risk score were independently associated with the risk of major coronary and vascular events, even after adjusting for multiple clinical factors. Those individuals whose 27-SNP risk score indicated a high genetic risk had a 1.65-fold increased hazard for major coronary events and a 1.37-fold increased hazard for major vascular events. Conversely, those whose genetic risk was intermediate had a 1.23-fold increased hazard for major coronary events and a 1.14-fold increased hazard for major vascular events [18]. The authors reported comparable results for individuals with high and intermediate genetic risk, as determined by the 6 million SNP score, whereby those classified as having a high genetic risk were associated with a 1.55-fold increased risk of major coronary events and a 1.31-fold increased risk of major vascular events, respectively [18]. Conversely, those classified as having an intermediate genetic risk were associated with 1.26-fold and 1.11-fold increased risk of major vascular events and major coronary events, respectively. Consequently, the 27-SNP score was equivalent to a 6 million SNP score in risk prediction [18].
To our best knowledge, this will be the first study examining the association between the PRS and CAD in the Polish population. The purpose of the LIPIDOGEN2015 study was to assess genetic risk factors associated with CAD and conditions that cause atherosclerosis. The LIPIDOGEN2015 population is distinctive in that it is representative of the Polish population in a primary care context, owing to its meticulous design. We are confident that by validating this PRS in the LIPIDOGEN2015 population, additional research can be conducted to examine the score’s applicability to other cohorts. Our study may also justify planning additional research, particularly randomized clinical trials (RCTs), in which patients are randomly assigned to receive pharmacological treatment for the prevention of CAD according to a PRS score adjustment for clinical variables. PRS stratification (together with/without the coronary artery calcium (CAC) score, currently increasingly being used [47, 49]) may help decide whether to start pharmacotherapy with statins.
In primary and secondary prevention, statins are the most effective therapy for reducing cholesterol levels and preventing CAD. Comparable across age groups, the quantity of statins necessary to avert a single cardiovascular death is equivalent in both primary and secondary prevention [15]. In addition, empirical studies have shown that PRS is a reliable method for predicting the prospective benefits of cholesterol-lowering drugs [16]. Klarin et al. propose that statins should be taken by people whose risk of developing ASCVD within 10 years is estimated at 5.0–19.9%. As an adjunct to conventional clinical risk assessments, PRS for CAD should be considered to be used in statin allocation. In epidemiological and clinical cohorts, high PRS for CAD is associated with incident events similar to most other risk factors [50].
In conclusion, the PRS scale developed for the Polish population, combined with clinical covariates, will facilitate the creation of an algorithm to predict long-term mortality. This will enable the earlier implementation of lifestyle changes and dietary adjustments and potentially initiate earlier pharmacotherapy for at-risk individuals. It is critically important especially in the high CVD risk population of Poland, with 80,000 MIs and 160,000 deaths due to cardiovascular disease annually, with only 24% of patients being on LDL-C goal, and less than 20% for those at very high CVD risk [51–55].