Introduction
Idiopathic pulmonary arterial hypertension (IPAH) is a rare and sporadic form of pulmonary arterial hypertension (PAH), which is characterized by elevated pulmonary arterial resistance leading to right heart failure [1]. However, IPAH is not associated with an underlying condition or family history. Idiopathic pulmonary arterial hypertension has a mean age of 35 ±15 years at diagnosis [2]. Over the past decades, the proportion of older patients diagnosed with idiopathic PAH has been increased by the PAH epidemiology [3, 4]. In addition, IPAH is characterized by a high burden of comorbidities [5, 6], such as obesity (30–38%), systemic hypertension (27–42%), type-2 diabetes mellitus (14%), and ischemic heart disease (10–12%).
The symptoms of IPAH are not specific, commonly include dyspnea, weakness, and recurrent syncope, and can be easily confused with many other, more common conditions [7] such as asthma, chronic obstructive pulmonary disease or sleep apnea; therefore, it is difficult to diagnose at an early stage. However, it is important to diagnose it earlier as it has been shown to be linked with better outcomes [8]. Due to complexity, a series of tests, such as electrocardiogram (ECG), echocardiogram, ventilation/perfusion scan (V/Q scan), pulmonary function tests, and right heart catheterization, are required to rule out other possible conditions, and confirm the diagnosis [9].
Pulmonary arterial hypertension patients could be treated by one of three relatively well-characterized pathways (nitric oxide, endothelin and prostacyclin), which are currently approved for PAH therapies [10]. To elucidate the complex pathogenic mechanisms that underlie this disease, an increasing number of researchers are conducting intensive research efforts. For example, Yu et al. [11] reported that TRPC channel overexpression may be partially responsible for the increased pulmonary arterial smooth muscle cell (PASMC) proliferation and pulmonary vascular medial hypertrophy in IPAH patients. Moreover, endothelin stimulates smooth muscle cell (SMC) proliferation via downstream signaling cascades involving endothelin type A and type B receptors [12]. Furthermore, inflammation has a key role in human PAH as well as in experimental models of pulmonary arterial hypertension [13–16]. However, the molecular mechanisms of PAH development are still not completely understood. In this study, we performed a systematic data analysis of key mRNAs and long non-coding RNA (lncRNAs) in peripheral blood mononuclear cells (PBMCs) of IPAH, which identified primarily dysregulated pathways, and highlighted key mRNAs and lncRNAs in PAH. The present study not only improved our understanding of the molecular mechanism, but also provided potential lncRNA biomarkers for further research. This way of bioinformation analysis has been widely applied in various disease fields [17–20].
Material and methods
Data collection and format conversion
Microarray-based gene expression data of 30 IPAH cases and 41 healthy donors were downloaded from the Gene Expression Omnibus (GEO, www.ncbi.nlm.nih.gov/geo/) database with accession number GSE33463 [21]. The gene expression data were median-normalized. The scaled intensity value was also log2-transformed.
Differential expression analysis
The gene expression data were used to identify differentially expression genes and lncRNAs by t-test. The differentially expressed genes/lncRNAs were identified at the threshold adjusted p-value < 0.05. The up- or down-regulation status was determined based on the fold change for each gene/lncRNA.
Gene Ontology and Kyoto Encyclopedia of Genes and Genomes enrichment analysis
The GO and KEGG enrichment analysis was implemented in javaGSEA (version 3.0) [22]. The genes were pre-ranked based their differential expression levels (t statistics). 10,000 permutations were used to calculate the enrichment significance.
Co-expression analysis of lncRNAs and PPI modules
Gene set enrichment analysis was used to evaluate the over-representation of the highly correlated genes with a given lncRNA in the PPI modules. The gene set enrichment analysis (GSEA) was implemented in the fgsea package with pre-rank mode in R programming language. The genes were ranked based on their correlation with each lncRNA.
Overrepresentation enrichment analysis
Overrepresentation enrichment analysis (ORA) was also implemented using the WEB-based Gene Set Analysis Toolkit (WebGestalt). The Gene Ontology [23] biological processes and KEGG pathways [24] were selected as the functional database.
Protein-protein interaction analysis
The Search Tool for the Retrieval of Interacting Genes/Proteins (STRING) [25] online software (https://string-db.org) was used to assess the interactions. The interactions of the proteins encoded by the differently expressed genes were searched using STRING online software. The PPI network was visualized using Cytoscape software (http://www.cytoscape.org). The Cytoscape MCODE [26] plug-in (version 3.4.0) was applied to search for modules with highly connected nodes from the PPI network. The resulting network was subjected to module analyses with the Plugin MCODE with the following parameters: degree cut-off ≥ 3, the nodes with edges ≥ 2-core, and number of nodes ≥ 7.
Results
Identification of differentially expressed mRNAs and lncRNAs in idiopathic pulmonary arterial hypertension
We first extracted the accession prefix from the RefSeq gene annotation to identify the lncRNA-related probes. The accession prefixes of NR and XR were used to identify the lncRNAs. In total, 48,803 probes were used to quantify the transcriptome, of which 1,155 lncRNAs were identified based on the accession prefixes. To identify the differentially expressed genes, we conducted differential expression analysis by comparing IPAH patients with healthy donors. A total of 3,134 genes, including 945 up-regulated and 2,189 down-regulated genes, were identified to be differentially expressed between the two groups (FDR < 0.05, Figure 1 A). Particularly, 9 up-regulated and 109 down-regulated lncRNAs were identified (Figure 1 B), the expression profiles of which showed a great difference between IPAH and healthy control groups.
Figure 1
Differentially expressed genes between idiopathic pulmonary arterial hypertension (IPAH) cases and healthy controls. The two heatmaps display the mRNA (A) and long non-coding RNA (lncRNA) (B) expression profiles. The expression patterns of both mRNAs and lncRNAs are significantly different between the IPAH cases and healthy controls
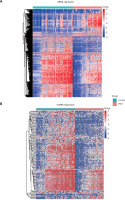
Gene Ontology and Kyoto Encyclopedia of Genes and Genomes enrichment analysis
To identify IPAH-related GO terms and KEGG pathways, we conducted gene set enrichment analysis. The GO analysis identified 442 up- regulated and 75 down-regulated GO terms (FDR < 0.05, Supplementary Table SI). The KEGG analysis also identified 24 up-regulated and 1 down-regulated KEGG pathways (FDR < 0.05, Supplementary Table SII).
Specifically, we identified establishment of protein localization to endoplasmic reticulum, protein localization to endoplasmic reticulum, translational initiation, nuclear transcribed mRNA catabolic process nonsense mediated decay, multi-organism metabolic process, RNA catabolic process, protein targeting to membrane, rRNA metabolic process, ribosome biogenesis, and alpha-beta T cell differentiation as the top-ten most significantly up-regulated GO terms (Figure 2 A). In contrast, we also identified regulation of T cell chemotaxis, gas transport, regulation of T cell migration, positive regulation of cellular extravasation, regulation of lymphocyte chemotaxis, oxygen transport, regulation of lymphocyte migration, regulation of monocyte chemotaxis, regulation of fever generation, and regulation of heat generation as the top-ten most significantly down-regulated GO terms (Figure 2 B). The up-regulated GO terms were primarily related to protein synthesis, RNA catabolic process and T cell differentiation. Particularly, a T cell induced severe inflammatory response may promote the occurrence of PAH. Moreover, the down-regulated GO terms could be summarized as the regulations of lymphocyte migration and chemotaxis, and fever or heat generation, suggesting that the down-regulation of lymphocyte mobility and fever or heat generation may be associated with PAH.
Figure 2
Gene set enrichment analysis (GSEA)-based Gene Ontology (GO) and Kyoto Encyclopedia of Genes and Genomes (KEGG) analysis. The enriched GO terms and KEGG pathways are presented in A and B, respectively. The grey and black bars represent the GO terms or KEGG pathways enriched by up-regulated or down-regulated genes, respectively
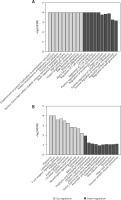
The top-ten most significant up-regulated KEGG pathways were ribosome, colorectal cancer, T cell receptor signaling pathway, prostate cancer, acute myeloid leukemia, circadian rhythm, endometrial cancer, neurotrophin signaling pathway, spliceosome, and basal cell carcinoma (Figure 2 C). In accordance with the up-regulated GO terms, the T cell receptor signaling pathway was also identified to be up-regulated by the KEGG pathway enrichment analysis. Although there was only one down-regulated KEGG pathway identified (Figure 2 D), the metabolism-related pathways accounted for 60% of the top-ten most significantly down-regulated KEGG pathways, indicating that the metabolism-related pathways played important roles in PAH.
Identification of modules in DEG-based PPI network
To construct the PPI network, we mapped the up- and down-regulated genes to the PPI network curated by the STRING database, respectively. The PPI network for the upregulated genes contained 443 nodes and 1,386 edges (Supplementary Table SIII). The nodes with the most connected adjacent nodes were deemed as hub nodes, and may have important functionality. We identified that OAS1 (2ʹ-5ʹ-oligoadenylate synthetase 1), GART (GAR transformylase), CXCL10 (C-X-C motif chemokine ligand 10), STAT1 (signal transducer and activator of transcription 1), and TLR4 (Toll like receptor 4) were the hub nodes in the up-regulated PPI network (connectivity degree > 30). It is worth noting that OAS1, CXCL10, STAT1 and TLR4 were the pro-inflammatory genes. The PPI network for the downregulated genes was constructed by 1,027 nodes and 6,625 edges, with hub nodes such as JUN and FOS (Supplementary Table SIV). Notably, JUN and FOS formed the AP-1 transcription factor, which was a negative regulator of cell differentiation [27].
Furthermore, to identify the modules in the DEG-based PPI network, we performed a module analysis of the network using the MCODE plugin (degree cut-off ≥ 3; the nodes with edges ≥ 2-core; number of nodes ≥ 7). We identified 7 up-regulated and 10 down-regulated modules in the PPI network, respectively. Particularly, the hub genes, such as OAS1, STAT1, TLR4, JUN and FOS, were also highlighted by the module analysis. In accordance with the GO and KEGG pathway enrichment analysis, the functional characterization of the PPI modules also revealed that pro-inflammatory pathways, such as the NOD-like receptor signaling pathway and chemokine signaling pathway, were highly enriched by the up-regulated modules based on over-representation enrichment analysis (ORA) (FDR < 0.05, Figure 3 A). The down-regulated modules were significantly enriched in Wnt signaling pathway, AP-1 transcription network, spliceosome, Th17 cell differentiation, RNA polymerase, ribosome, and ubiquitin mediated proteolysis (FDR < 0.05, Figure 3 B).
Figure 3
The up- and down-regulated PPI modules. The up- and down-regulated PPI modules are illustrated in A and B, respectively. The nodes with red and blue colors represent the up- and down-regulated genes. The PPI modules are characterized by Gene Ontology (GO) terms or Kyoto Encyclopedia of Genes and Genomes (KEGG) pathways based on overrepresentation enrichment analysis (ORA)
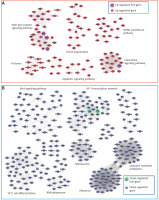
Co-expression analysis of lncRNAs and PPI modules
To link the differentially expressed lncRNAs with the PPI modules, we first calculated the correlation coefficient between each lncRNA and mRNA pair. Subsequently, GSEA was performed to evaluate the enrichment degree of the highly correlated genes for each lncRNA in each module. We identified 18 pairs of lncRNA and PPI modules, including 7 lncRNAs and 4 PPI modules (adjusted p-value < 0.01). Interestingly, all 7 lncRNAs, i.e. LOC643888, LOC554206, IL8RBP, LOC642897, SLC6A10P, RPL23AP7 and LOC400759, were up-regulated in IPAH samples (Figure 4 A). The mRNA and lncRNA pairs were present in Figures 4 B and 4 C, which showed that the lncRNAs had a high degree of connectivity in the network. The two lncRNAs, LOC643888 and LOC554206, were directly connected with purinergic receptors, such as P2RY12, P2RY13 and P2RY14, and C-C motif chemokine receptors, such as CCR2 and CCR5 (Figure 4 B). In contrast, among the seven lncRNAs, six were highly correlated with most of the genes involved in the other PPI module (Figure 4 C), indicating that these lncRNAs were closely associated with the PPI module.
Figure 4
The expression patterns of seven key long non-coding RNAs (lncRNAs). The expression patterns of the seven lncRNAs in idiopathic pulmonary arterial hypertension (IPAH) and healthy controls are illustrated in A. The grey and black boxes indicate the IPAH and healthy control groups, respectively
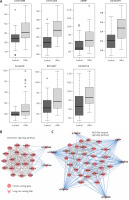
Moreover, the GSEA based on the two PPI modules, which were characterized by pro-inflammatory pathways, the chemokine signaling pathway (Figures 5 A, B) and the NOD-like receptor signaling pathway (Figures 5 C–H), further demonstrated that these lncRNAs played critical roles in the pathogenesis of IPAH by participating in the pro-inflammatory pathways (adjusted p-value < 0.05).
Figure 5
The gene set enrichment analysis of the pro-inflammatory pathway-related long non-coding RNA (lncRNA)-module pairs. Two lncRNAs, LOC554206 (A) and LOC643888 (B), are associated with modules characterized by the chemokine signaling pathway. Moreover, 6 other lncRNAs (IL8RBP, LOC400759, LOC554206, LOC642897, LOC643888 and SLC6A10P) are associated with NOD-like receptor signaling pathway (E–H)
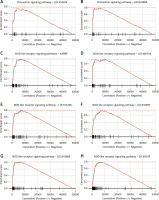
Discussion
Pulmonary arterial hypertension is a progressive disease of various origins, which has a poor prognosis and affects more than 100 million people worldwide [28]. Idiopathic pulmonary arterial hypertension is defined as a rare subgroup of PAH, in which there is neither a family history of, nor an identified risk factor, for PAH.
In this study, we aimed to analyze the key mRNAs and lncRNAs in IPAH. To explore the molecular mechanism of IPAH, we collected gene expression datasets of PBMCs from IPAH cases and healthy donors. To identify the probes on the array corresponding to lncRNAs sequences, we identified a total of 1,155 lncRNAs based on the accession prefixes of NR and XR.
To investigate the biological differences between IPAH cases and healthy controls, we performed differential expression analysis of the gene expression data, and identified a total of 3,134 genes, including 945 up-regulated and 2,189 down-regulated genes, 118 of which were lncRNAs. Subsequently, the differentially expressed mRNAs were subjected to GO and KEGG enrichment analyses. The GO analysis identified 442 up-regulated and 75 down-regulated GO terms (FDR < 0.05, Supplementary Table SI). The KEGG analysis also identified 24 up-regulated and 1 down-regulated KEGG pathways (FDR < 0.05, Supplementary Table SII). The GO and KEGG enrichment analysis of the up-regulated genes revealed that the T cell receptor signaling pathway and T cell differentiation played key roles in the occurrence of IPAH. Moreover, the down-regulated GO terms could be summarized as the regulations of lymphocyte migration and chemotaxis, and fever or heat generation, suggesting that the down-regulation of lymphocyte mobility and fever or heat generation may be closely associated with PAH.
To investigate the interactions between the proteins encoded by the DEGs, we mapped the DEGs to the PPI network. We observed that OAS1, CXCL10, STAT1 and TLR4 were key components in the modules of the up-regulated PPI network. Further functional characterization of these PPI modules revealed that pro-inflammatory pathways, such as the NOD-like receptor signaling pathway and chemokine signaling pathway, were highly enriched by the up-regulated modules based on over-representation enrichment analysis (ORA) (FDR < 0.05, Figure 3 A).
To predict the potential functional roles of the differentially expressed lncRNAs, the Spearman correlation coefficient for lncRNA-DE-mRNA pairs was calculated to identify the modules with a high correlation with each lncRNA. Seven lncRNAs, i.e. LOC643888, LOC554206, IL8RBP, LOC642897, SLC6A10P, RPL23AP7 and LOC400759, showed a high correlation with PPI modules. Notably, 6 of these lncRNAs were associated with modules characterized by the NOD-like receptor signaling pathway and chemokine signaling pathway.
However, there are some limitations to this study. For example, the functional annotation of the lncRNAs by co-expression and PPI analysis can only suggest that the lncRNAs may participate in the subnetwork or pathway, while the specific regulatory relationships between the lncRNAs and protein-coding genes are still unknown, which requires more experiments to validate these interactions.
In conclusion, our integrative analysis revealed key mRNAs and lncRNAs, involved in IPAH. The results not only identified IPAH-related pro-inflammatory pathways such as the NOD-like receptor signaling pathway and chemokine signaling pathway, and lncRNAs, but also improved our understanding of the molecular mechanism for the occurrence of IPAH.