Introduction
Osteoporosis (OP) is a metabolic bone disease characterized by low bone mass and alterations in bone microstructure, leading to increased bone fragility and a higher risk of fractures [1]. Postmenopausal osteoporosis (PMO) is a prevalent skeletal disorder in postmenopausal women [2, 3]. This systemic disease is featured by the deficiency of bone mass and elevated bone fracture risk [4]. Ageing, estrogen deficiency, prolonged calcium deficiency, and smoking are strong independent risk factors for PMO [5, 6]. The alleviation of complications associated with PMO is a challenging task to obtain [7, 8]. Of note, the imbalance between the osteogenic and osteoclastic activities is a contributor to osteoporosis [9]. Mesenchymal stem cells (MSCs) are regarded as multipotent cells which can differentiate into osteoblasts, but this compromised ability can cause bone disorders, including osteoporosis [10]. Reduced osteogenesis can be observed in bone marrow mesenchymal stem cells (BMSCs) in patients with osteoporosis [11]. In postmenopausal women, insufficient bone formation by osteoblasts from BMSCs is responsible for osteoporosis [12]. Therefore, it is significant to seek novel targets [13] for regulating osteoblast osteogenesis to treat PMO.
In the present study, weighted gene co-expression network analysis (WGCNA) combined with GEO database-based differential analysis and least absolute shrinkage and selection operator (LASSO) regression analysis identified phosphatidylinositol-4,5-bisphosphate 3-kinase catalytic subunit α (PIK3CA) as the core gene of the PI3K-Akt pathway affecting PMO. PIK3CA can encode the p110α catalytic subunit of PI3K and assist the PI3K/Akt pathway to regulate important cellular functions [14]. Of note, PIK3CA was identified as a hub protein that might have an impact on the progression of osteoporosis [15]. PIK3CA/Akt/GSK3β/β-catenin signaling could be activated by icariin, thereby supporting the protection against glucocorticoid-induced osteoporosis [16]. It should be noted that our bioinformatics analysis screened Kruppel-like factor 2 (KLF2) as the potential regulator of PIK3CA. KLF2 is recognized as a zinc finger transcription factor [17]. As previously reported, KLF2 expression could be increased by BMSC-derived extracellular vesicles (EVs) carrying miR-15b, which facilitated osteoblast differentiation [18]. KLF2 downregulation could elevate osteoclast differentiation while suppressing osteoblast differentiation [19]. KLF2 expression could be induced by anti-resorptive bisphosphonates, which are applied for treating osteoporosis [20]. Accumulating evidence has suggested the interaction between KLF2 and the PI3K-Akt pathway [21, 22]. Activation of the PI3K/Akt pathway was suggested to be involved in osteoblast differentiation of MSCs [23]. Strikingly, a previous study revealed that activation of the PI3K-Akt pathway due to miR-483-5p silencing could ameliorate PMO [24].
Given the reports mentioned above, we hypothesized in the present study that the BMSC-secreted transcription factor KLF2 might affect osteoblast differentiation of BMSCs in PMO by regulating PIK3CA and the PI3K-Akt pathway. This study sheds light on the potential of MSCs in managing PMO through the identification of molecular mechanisms regulating osteoblast differentiation.
Material and methods
GEO microarray data acquisition
Osteoporosis expression profile datasets GSE7158 and GSE56815, and the BMSC-related dataset GSE105145 were obtained through the GEO database. Among them, the GSE7158 dataset included 14 normal control samples and 12 osteoporosis patient samples, the GSE56815 dataset included 20 normal control samples and 20 osteoporosis patient samples, and the GSE105145 dataset included 3 samples of BMSCs and 3 dental pulp MSC samples.
Datasets GSE7158 and GSE56815 were further merged and the ComBat function of the package sva was used to correct batch effects. All analyses in this paper were performed under R version 4.2.1.
Differential gene expression analysis
Differential analysis was performed through the “limma” package of R language, selecting the differentially expressed genes (DEGs) in each dataset with |logFC| > 1 and p < 0.05 as the screening condition. Volcano maps were drawn using the “ggplot2” package of R language and differential gene expression heat maps were drawn using the “heatmap” package.
WGCNA
To further understand the interrelationship between the merged genes from the GSE7158 and GSE56815 datasets, and to identify the central genes of the gene modules, the co-expression network of osteoporosis samples was constructed with the WGCNA algorithm. The sample cluster analysis was performed using the R language “hclust” package. Gene co-expression networks were constructed using the R language “WGCNA” package. First, the expression of individual genes was transformed into a similarity matrix based on the Pearson correlation coefficient between pairs of genes. A hierarchical clustering dendrogram was constructed using an expression matrix to detect outliers. Then, the scale-free topological fitting exponent was calculated as a function of soft threshold power based on the network topological analysis function. The topological overlap matrix-based dissimilarity measure was then used to calculate the connectivity between genes in the module with similar expression profiles, and hierarchical clustering was utilized to produce a hierarchical clustering dendrogram of genes. Finally, a dynamic mixed cutting method was used to divide these genes into different modules.
Gene selection by GeneCards analysis
Genes associated with “PMO” and “osteoblast differentiation” were searched by the GeneCards database and ranked by the Relevance score from high to low. The selected genes were imported into the STRING database for protein-protein interaction (PPI) analysis, where the “species” was limited to “human”. The PPI network of regulatory relationship was drawn through the Cytoscape software (v3.6.0).
Gene functional enrichment analysis
The analysis results were intersected using the R language “VennDiagram” package, and a Venn diagram of the intersected DEGs was obtained. GO (biological processes, cellular components, and molecular functions) and KEGG enrichment analyses were performed using the R language “ClusterProfiler” package. The cellular functions and pathways mainly affected by potential targets and key targets were analyzed. p < 0.05 was considered statistically significant.
Lasso regression analysis
Regression analysis is a mathematical model that constructs quantitative relationships between variables based on determining the dependent and independent variables. The “glmnet ()” function package in R Studio software was called to load the candidate gene matrix. When α = 1, the appropriate λ value was selected, and the internal validation was implemented by the ten-fold cross-validation method to determine the best model.
M – number of samples, k – number of parameters, λ – regularization parameters.
Receiver operating characteristic (ROC) analysis
The “pROC ()” function in the R Studio software was used to draw the ROC curve for the model. The overall survival (OS) included in the data was selected as the dependent variable. With the specificity as the horizontal axis and the sensitivity as the vertical axis, the ability of the AUC evaluation model to distinguish the risk of PMO prognosis was calculated. In the case of AUC > 0.5, the closer the AUC was to 1, the better the model could predict the PMO prognosis.
Cell culture and treatment
Human bone marrow mesenchymal stem cell line UE7T-13 (Biobw, Beijing, China) was cultured in RPMI-1640 medium (11875093, Gibco) containing 10% fetal bovine serum (FBS; 10099141, Gibco), and 1% penicillin/streptomycin (SV30010, Gibco) at 37°C under 5% CO2. Then cells were transfected with siRNA plasmids (HanBio, Shanghai, China) targeting PIK3CA (PIK3CA-si) and KLF2 (KLF2-si) as well their corresponding NC (PIK3CA-si-NC and KLF2-si-NC) using Lipofectamine 3000 reagents (L3000015, Invitrogen). The normal cells were cultured with normal culture medium while the remaining cells were cultured with the osteogenic induction medium (#HUXMX-90021, Cyagen Bioscience Inc., Suzhou, Jiangsu, China). siRNA sequences are shown in Supplementary Table SI.
Cell differentiation determination
To induce osteoblast differentiation, BMSCs (1 × 105) were cultured in a endothelial cells (ECs) were cultured on 0.1% gelatin-coated coverslips in 24-well culture plates and osteogenic induction medium (HUXMX-90021, Cyagen) for 1 week. Alkaline phosphatase (ALP) staining and alizarin red-S (ARS) staining were carried out using an Alkaline Phosphatase Assay Kit (P0321S, Beyotime, Shanghai, China) and alizarin red-S solution (C0148S, Beyotime), respectively.
RT-qPCR
Total RNA was extracted from cells and tissues via homogenization in TRIzol reagent (10296010, Thermo Fisher Scientific Inc., Waltham, MA; 1 ml per 100 mg tissues), addition of chloroform (200 ml), and centrifugation at 12000 g and 4°C for 10 min. The upper aqueous phase was collected and mixed with 500 ml of isopropanol to precipitate the RNA. RNA was dissolved in RNase-free water (10–30 ml) and quantified on a NanoDrop ND-3300 Spectrophotometer (Thermo Fisher Scientific Inc.). The RNA (1 mg) was reverse transcribed using TaqMan Reverse Transcription Kit (N8080234, Thermo Fisher Scientific Inc.), and qPCR was performed using PowerUp SYBR Green Master Mix (Thermo Fisher Scientific Inc., A25741). GAPDH and U6 were regarded as internal references and the fold changes were calculated by relative quantification (2-ΔΔCt method). The primer sequences are shown in Supplemnetary Table SII.
Western blot
Total protein was extracted, separated and transferred onto membranes. The membrane was blocked using TBST (5 ml) with 5% skimmed milk powder (P0216-300 g, Beyotime) and underwent overnight incubation at 4°C with primary antibodies to PIK3CA (p110 & p85) (51 kDa, #ab40776, Abcam, Cambridge, UK), KLF2 (139 kDa, #ab236507, Abcam), Akt1 (56 kDa, #ab238477, Abcam), p-Akt1 (56 kDa, #ab81283, Abcam), collagen I (139 kDa, #ab138492, Abcam), ALP (39 kDa, #ab307726, Sigma), RUNX2 (57 kDa, #ab236639, Abcam), OCN (11 kDa, #ab133612, Abcam), and GAPDH (36 kDa, #PA1-987, Thermo Fisher Scientific Inc.). The next day, the membrane was incubated with HRP-labeled secondary antibody goat anti-rabbit (A0208, Beyotime). ECL (P0018FS, Beyotime) was used for chemiluminescence detection and membranes were photographed by the ChemiDoc XRS + system (Bio-Rad). The band intensity was quantified using Image J analysis software, normalized to GAPDH [25].
ChIP assay
BMSCs cultured in the osteogenic induction medium for 1 week were collected and probed with antibodies to KLF2 (139 kDa, #ab236507, Abcam) and IgG (52 kDa, #ab109489, Abcam, serving as a NC). The primers used for this assay are shown in Supplementary Table SIII [26].
Statistical analysis
The Wilcoxon test was used to compare differences between the two sets of non-normally distributed data. The unpaired t-test was used to compare differences between two sets of normally distributed data. The Pearson correlation analysis was performed to compare the correlation between the two data groups and to calculate the correlation coefficients. The R software (4.2.1), SPSS (22.0), and Prism were used for statistical analysis and mapping. p < 0.05 demonstrated that the difference was statistically significant.
Results
GEO-based differential analysis for screening DEGs in PMO
In order to find the molecular mechanisms to alleviate PMO, differential analysis and WGCNA through GSE7158 and GSE56815 datasets were performed. In combination with the GeneCards website, Lasso regression analysis and ROC curve analysis were carried out, and the biological function of core genes was analyzed by functional enrichment. The BMSC-related dataset GSE105145 was used to screen the transcription factors involved in the regulation, and a PPI regulatory network was constructed to provide a theoretical basis for further understanding the mechanism of PMO and finding potential diagnosis and treatment targets. The bioinformatics analysis process is shown in Supplementary Figure S1.
To screen DEGs in PMO, differential analysis was performed using the combined dataset of GSE7158 and GSE56815. A total of 10419 DEGs were selected, where 8308 DEGs were downregulated, and 2112 DEGs were upregulated (Figures 1 A, B).
WGCNA-based screening for the green core module
First, 12530 genes in the expression profiles of the combined dataset of GSE7158 and GSE56815 were selected for cluster evaluation, and a total of 66 samples were used for subsequent WGCNA (Figure 2 A). The best value of the soft threshold (power) was set to 14 to build a gene expression network (Figure 2 B).
Figure 2
Data validation and screening for optimal soft thresholds. A – Heterogeneity viewed by sample clustering. B – Non-scale fitting index analysis of soft threshold. C – Analysis of soft threshold average connectivity
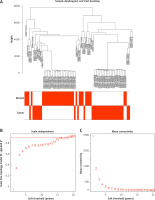
Modules with high similarity of module eigengenes were merged by dynamic mixed clipping method, where the minModules size was set to 30, deepSplit to the default value of 2, and cut height to 0.25. Each module was shown in a different color (Figure 3 A). Pearson correlation analysis was performed between each module eigengene and different groups (Figure 3 B). The six co-expression modules with the top absolute value of the correlation coefficient and p value were selected for subsequent analysis, including the green module (2647 genes), the black module (4413 genes), the purple module (99 genes), the magenta module (241 genes), the pink module (824 genes) and the grey module (4281 genes). Among them, the green module showed the most significant correlation (cor = 0.76, p < 1e-200) (Figure 3 C).
Identification of 197 core DEGs related to PMO
GEO-based differential analysis was performed to screen PMO-related DEGs. The WGCNA screened core green module-related DEGs for Venn analysis, and 2189 common DEGs (Figure 4 A) were obtained. Through the GeneCards website, 1188 PMO-related genes were further screened to intersect with 2189 DEGs, and 197 candidate DEGs related to PMO progression were obtained (Figure 4 B).
Figure 4
Screening for core DEGs and regulatory function analysis. A – Venn diagram of the green module-related DEGs and DEGs in GEO database. B – Venn diagram of PMO-related genes and 2189 DEGs. C – KEGG pathway enrichment analysis of candidate DEGs. D–F – Functional analysis of candidate DEGs in biological processes (D), cellular components (E), and molecular functions (F)
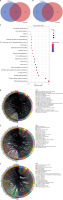
The candidate DEGs were subjected to KEGG and GO functional enrichment analyses. The results of the KEGG pathway analysis showed that the candidate DEGs were mainly enriched in the PI3K-Akt pathway, cytokine-cytokine receptor interaction, Jak-STAT pathway, and Th17 cell differentiation pathway (Figure 4 C). The results of GO functional analysis revealed that the candidate DEGs were mainly enriched in ossification, response to peptide, organic hydroxy compound metabolic process and multicellular organismal homeostasis in biological processes. The candidate DEGs were mainly enriched in the collagen-containing extracellular matrix, extracellular matrix, blood microparticle, and secretory granule lumen in cellular components. The candidate DEGs were mainly enriched in receptor regulator activity, receptor ligand activity, growth factor activity, and cytokine receptor binding in molecular function (Figures 4 D–F).
The above results suggested that the candidate DEGs mainly played roles in ossification and cytokine regulation, and were enriched in extracellular matrix and vesicles. The molecular functions of candidate DEGs were mainly related to cytokine-related enzyme activity and receptor activity. According to the results of the KEGG analysis, the candidate DEGs were involved in the regulation of the PI3K-Akt pathway. It has been found that the activation of the PI3K-Akt pathway could regulate the bone balance of PMO, inhibit osteoblast apoptosis and promote cell proliferation [27]. Therefore, we further investigated the molecular regulatory mechanism of the PI3K-Akt pathway in PMO.
PIK3CA as the core gene of the PI3K-Akt pathway affecting PMO
To further investigate the core regulatory genes in candidate DEGs, we performed Lasso regression analysis of the 14 related genes involved in the PI3K-Akt pathway (GHR, ATF2, IL4R, COL2A1, IRS1, FGF18, JAK2, CSF1, HSP90AA1, FLT3, MAPK3, PIK3CA, CCND1, and MTOR) in the candidate DEGs. The Lasso algorithm used the L1 criterion for model construction, and the change of the regression coefficient under the L1 criterion is shown in Figure 5 A. With a parameter α = 1, ten-fold cross-validation was used for model internal validation. The selection process diagram of the cross-validation parameter λ was drawn, where the log (λ) was indicated on the horizontal axis and the root mean square error value (RMSE) on the vertical axis (Figure 5 B). When the model variable value was 4, the RMSE value was minimal; therefore, the corresponding candidate DEGs (PIK3CA, IL4R, JAK2, and MAPK3) were selected for subsequent analysis.
Figure 5
Screening for the core genes of the PI3K-Akt pathway affecting PMO. A – Lasso regression analysis of candidate DEGs and regression coefficient variation diagram under L1 criterion. B – Selection process diagram of cross-validation parameter λ. C – ROC curve diagram of 4 candidate DEGs. D – Boxplot of the expression of 4 candidate DEGs in the combined dataset of GSE7158 and GSE56815. E – Construction of a PPI network of the top 30 genes related to osteoblast differentiation retrieved from the GeneCards website and core genes of the PI3K-Akt pathway. F – Calculation of the degree value of genes in the PPI network
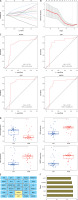
The ROC curves of the four DEGs were drawn, and AUC values were calculated (Figure 5 C). The results showed that the AUC values of PIK3CA, IL4R, JAK2, and MAPK3 were 0.754, 0.787, 0.793, and 0.792, respectively, all of which met AUC > 0.75, suggesting that the model had a good discriminatory ability to predict PMO prognosis. Further calculation of PIK3CA, IL4R, JAK2, and MAPK3 expression in PMO showed that PIK3CA was significantly less expressed in the disease group than in the control group. In contrast, the other three genes showed the opposite trend (Figure 5 D). Therefore, we selected PIK3CA as the core gene to explore the regulatory mechanism of the PI3K-Akt pathway in PMO.
Previous literature has shown that promoting osteoblast differentiation of BMSCs can effectively alleviate PMO [28]. In order to further explore the mechanism, we screened for genes related to osteoblast differentiation through the GeneCards website, and selected the top 30 genes and core genes of the PI3K-Akt pathway to construct a PPI network (Figure 5 E). From the network, we observed that Akt1 and PIK3CA, the core genes of the PI3K-Akt pathway, were closely related to osteoblast differentiation. Further calculation of the Degree value, which is the most direct measure to characterize node centrality in a network analysis, identified Akt1 to be one of the core genes in the PPI network (Figure 5 F). The above results indicate that the PI3K-Akt pathway can participate in the osteoblast differentiation of BMSCs.
PIK3CA promoted osteoblast differentiation of BMSCs
Having identified PIK3CA as the core gene of the PI3K-Akt pathway affecting PMO, we continued to verify the effect of PIK3CA on the osteoblast differentiation of BMSCs. The mRNA and protein expression of PIK3CA was found to be increased in the BMSCs cultured with osteogenic induction medium versus normal BMSCs (Figures 6 A, B), implying that PIK3CA may facilitate osteoblast differentiation of BMSCs. Subsequently, we designed two siRNA sequences targeting PIK3CA and transfected them into BMSCs. The PIK3CA-si2 sequence showed superior silencing efficiency and was used for subsequent experiments (Figures 6 C, D).
Figure 6
PIK3CA facilitates osteoblast differentiation of BMSCs. A – mRNA expression of PIK3CA in the BMSCs cultured with normal medium and osteogenic induction medium detected by RT-qPCR. B – Western blot of PIK3CA protein in the BMSCs cultured with normal medium and osteogenic induction medium. C – Silencing efficiency of PIK3CA-si in the BMSCs detected by RT-qPCR. D – Silencing efficiency of PIK3CA-si in the BMSCs detected by Western blot. E – Osteoblast differentiation of BMSCs transfected with PIK3CA-si detected by ALP staining and ARS staining. F – Western blot of osteoblast differentiation-related proteins collagen I, ALP, RUNX2 and OCN was decreased in BMSCs transfected with PIK3CA-si. G – Phosphorylation level of Akt1 in BMSCs transfected with PIK-3CA-si detected by Western blot. *p < 0.05, ** p < 0.01
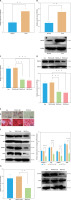
As shown in Figure 6 E, a reduction was noted in the osteoblast differentiation of BMSCs transfected with PIK3CA-si. In addition, the expression of osteoblast differentiation-related proteins collagen I, ALP, RUNX2 and OCN was decreased in BMSCs transfected with PIK3CA-si (Figure 6 F). Meanwhile, silencing of PIK3CA inhibited the phosphorylation level of Akt1 (Figure 6 G). Altogether, these results demonstrate that PIK3CA may be a key gene to facilitate osteoblast differentiation of BMSCs.
The transcription factor KLF2 promoted PIK3CA transcription
The transcription factor is a key molecule of gene expression regulation. To explore whether there was a molecular mechanism of the transcription factor to regulate PIK3CA expression in PMO, 1369 human transcription factors were downloaded through the humantfs website to intersect with the top 100 DEGs in PMO, and only the transcription factor KLF2 was obtained (Figure 7 A). Pearson correlation analysis results showed that in the combined dataset of GSE7158 and GSE56815, transcription factor KLF2 was positively correlated with PIK3CA expression (Figure 7 B). Using the JASPAR website, the binding site of the transcription factor KLF2 (Figure 7 C) and that between KLF2 and the PIK3CA promoter region (Supplementary Table SIV) were analyzed.
Figure 7
Screening for transcription factors regulating PIK3CA expression. A – Venn diagram of DEGs in PMO and human transcription factors. B – Pearson correlation analysis of transcription factor KLF2 and PIK3CA. C – JASPAR website was used to predict the binding site of transcription factor KLF2. D – Heat map of DEGs in the BMSC-related GSE105145 dataset. E – Volcano map of DEGs in the BMSC-related GSE105145 dataset. F – Expression of transcription factor KLF2 in GSE105145. *p < 0.05 vs. the control group
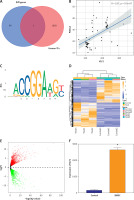
BMSCs can self-renew and multi-differentiate, and they are indispensable components in osteoblast differentiation and skeletal remodeling in PMO. The process is controlled by a multistep molecular pathway, and regulated by different transcription factors and signaling proteins. To explore the expression of the transcription factor KLF2 in BMSCs, we performed a differential analysis of the BMSC-related dataset GSE105145 and selected 4510 DEGs, including 2369 upregulated genes and 2141 downregulated genes (Figures 7 D, E). We found that the transcription factor KLF2 was significantly highly expressed in the BMSC samples, compared to that in the control samples (Figure 7 F). It has been well documented that KLF2 promoted the stemness maintenance and self-renewal of BMSCs [29]. Therefore, we could draw the preliminary conclusion that BMSCs might participate in the transcription and development of PMO by regulating PIK3CA transcription through secretion of the transcription factor KLF2.
KLF2 was involved in the osteoblast differentiation of BMSCs by regulating the PI3K-Akt pathway
To verify whether the transcription of PIK3CA is regulated by KLF2, we first detected the expression of KLF2 in BMSCs cultured with osteogenic induction medium by RT-qPCR and Western blot. The results showed an increase of the mRNA and protein expression of PIK3CA in the BMSCs cultured with osteogenic induction medium versus normal BMSCs (Figures 8 A, B). Then, we designed two siRNA sequences targeting KLF2 and transfected them into BMSCs. RT-qPCR and Western blot identified their silencing efficiency, with the KLF2-si1 sequence showing higher silencing efficiency, and it was used for subsequent experiments (Figures 8 C, D).
Figure 8
KLF2 enhances the osteoblast differentiation of BMSCs by regulating the PI3K-Akt pathway. A – mRNA expression of KLF2 in BMSCs cultured with normal medium and osteogenic induction medium detected by RT-qPCR. B – Western blot of KLF2 protein in BMSCs cultured with normal medium and osteogenic induction medium. C – Silencing efficiency of KLF2-si in BMSCs detected by RT-qPCR. D – Silencing efficiency of KLF2-si in BMSCs detected by Western blot. E – Osteoblast differentiation of BMSCs transfected with KLF2-si detected by ALP staining and ARS staining. F – Western blot showing that expression of osteoblast differentiation-related proteins collagen I, ALP, RUNX2 and OCN was decreased in BMSCs transfected with KLF2-si. G – PIK3CA protein expression and phosphorylation level of Akt1 in BMSCs transfected with KLF2-si detected by Western blot. H – Binding of KLF2 to the promoter region of PIK3CA analyzed by ChIP assay. *p < 0.05, **p < 0.01.
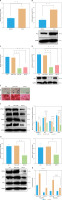
In the absence of KLF2, osteoblast differentiation of BMSCs (Figure 8 E) was weakened in addition to decreased expression of osteoblast differentiation-related proteins collagen I, ALP, RUNX2 and OCN (Figure 8 F). Meanwhile, silencing of KLF2 inhibited the phosphorylation level of Akt1 (Figure 8 G). Further, ChIP results demonstrated that KLF2 could bind to the promoter region of PIK3CA (Figure 8 H), indicating that the transcription of PIK3CA is regulated by KLF2. Overall, KLF2 can regulate the PI3K-Akt signaling pathway through PIK3CA, thereby promoting the osteoblast differentiation of BMSCs.
Discussion
Postmenopausal-triggered bone loss is mainly attributed to declined core transcription factors of BMSCs [30]. The therapeutic strategy by enhancing osteoblast differentiation is considered as a promising direction for osteoporosis treatment. Herein, we illustrated the mechanistic basis for the anti-osteoporotic effect of BMSCs using bioinformatics and experimental analyses. The study identified the core gene PIK3CA involved in regulation of the PI3K-Akt pathway in PMO, and its expression was beneficial for the osteoblast differentiation of BMSCs, regulated by the transcription factor KLF2. The findings provide a starting point for future research on MSC-based therapies to treat PMO.
We performed differential analysis to screen for DEGs in PMO, and WGCNA singled out a green core module. A total of 197 genes were obtained as candidate ones in PMO, and combined with GO and KEGG enrichment analyses, PIK3CA was further identified as the core gene of the PI3K-Akt pathway for affecting PMO. Notably, PIK3CA was reported to be a pivotal protein that might regulate osteoporosis progression [15]. PIK3CA could be regulated by Jiawei Buguzhi Pills and could play a therapeutic role in PMO [31]. Activated PIK3CA/Akt/GSK3β/β-catenin signaling by icariin could exert a protectory function against glucocorticoid-induced osteoporosis [16]. Additionally, PIK3CA activation mutation could augment osteoblast differentiation in macrodactyly-derived BMSCs by regulating the PI3K/Akt/mTOR pathway [32]. These studies can provide support for our findings regarding the potential role of PIK3CA in PMO.
We further found in the present study that BMSCs could secrete the transcription factor KLF2 to increase the transcription of PIK3CA and enhance osteoblast differentiation of BMSCs. KLF2 can maintain the stemness of MSCs in bone regeneration [29] and result in the self-renewal of human BMSCs [33]. Prior research demonstrated that the culture of human BMSCs with the medium could increase the expression of KLF2 [34]. Intriguingly, KLF2 expression can be elevated by BMSC-derived EVs carrying miR-15b, which facilitates osteoblast differentiation [18]. Moreover, the p53/KLF2 axis dependent on JMJD3 was found to be activated in the presence of BMSC-derived EVs, thereby alleviating hypoxic-ischemic brain injury in neonatal mice [35]. To our knowledge, the regulatory effect of KLF2 on the PI3K-Akt pathway has been previously revealed. For instance, resveratrol-regulated KLF2 expression activates the PI3K/Akt pathway, protecting Min6 cells against uric acid-induced injury [36]. Unlike our results, the previous research failed to investigate the direct regulatory effect of KLF2 on PI3KCA. The function of KLF2 in osteoblast differentiation has been increasingly highlighted. Overexpressed KLF2 in MC3T3-E1 cells results in increased levels of osteoblast differentiation markers, including Alp, Osx, and Ocn, and regulates RUNX2 to facilitate osteoblast differentiation [37]. Additionally, KLF2 demethylation by Kdm3a stimulates osteoblast differentiation in osteoporosis [38]. Therefore, BMSC-secreted KLF2 could activate the PIK3CA-mediated PI3K-Akt pathway to promote osteoblast differentiation in PMO.
Furthermore, our study demonstrated that the PI3K-Akt pathway participated in osteoblast differentiation in PMO. QingYan formula extracts had a potential alleviatory effect on PMO, which was achieved partially by activating the PI3K-Akt pathway in osteoblasts [39]. Intriguingly, the activated PI3K-Akt pathway suppresses chaperone-mediated autophagy activity of rat BMSCs, thereby promoting the osteoblast differentiation of rat BMSCs [40]. Furthermore, upregulated expression of the PI3K-Akt pathway-related genes by beraprost aids in enhancing the differentiation of mouse BMSCs to ameliorate PMO [41]. Inhibition of the PI3K-Akt pathway by miR-320a can alleviate the development of PMO [42]. The results of these reports are all consistent with ours, showing the participation of the PI3K-Akt pathway in osteoblast differentiation in PMO.
Taken together, the results of the present study demonstrate that BMSCs effectively alleviated PMO by secreting the transcription factor KLF2, which increased PIK3CA expression and activated the PI3K-Akt pathway to promote osteoblast differentiation. The study provides a foundation for future research on the role of PIK3CA and KLF2 in promoting osteoblast differentiation in MSCs and their potential in developing novel therapeutic targets. The study also highlights the importance of investigating the underlying molecular mechanisms in the use of MSCs as a source of cell-based therapy for PMO. However, the study has certain limitations such as its focus on molecular mechanisms only, absence of clinical trials, and lack of investigation of other transcription factors and their interaction with PIK3CA. Future studies could focus on exploring the potential of MSC-based therapy in clinical trials and identifying novel targets for osteoblast differentiation, e.g., targeting other transcription factors that interact with PIK3CA. Additionally, further research could aid in identifying potential strategies to optimize this therapy for patients with osteoporosis.