Introduction
Pancreatic ductal adenocarcinoma (PDAC) accounts for about 90% of pancreatic cancer. Pancreatic ductal adenocarcinoma is one of the most aggressive cancers with an estimated 367,000 new cases diagnosed and 359,000 associated deaths worldwide in 2015 [1, 2]. Although researchers have paid attention to exploring novel prognostic and therapeutic targets of PDAC, the 5-year survival rate for PDAC is as low as 5% [3, 4]. Moreover, the molecular mechanisms underlying PDAC remain unclear [5]. Previous studies showed that genetic alterations and non-coding RNAs were associated with PDAC progression. For example, more than 90% of PDAC cases were identified with oncogenic KRAS mutation, and TP53 mutation (75%), SMAD4 mutation (22%), and CDKN2A/B mutation (18%) were also widely observed in PDAC tumors. Meanwhile, a series of miRNAs, including miR-34a, miR-506, and miR-494, played an important role in PDAC growth and autophagy. Identification of novel regulators that contribute to pancreatic cancer progression will be helpful to develop novel therapeutic approaches [6].
More and more studies have showed that 80–90% of RNA molecules were non-coding RNAs in human cells [7]. Many kinds of ncRNAs, including miRNAs, lncRNAs and small nucleolar RNAs (snoRNAs), act as important regulators of cancer progression. Of these, miRNA is the most well-known post-transcriptional small non-coding RNA (20–22 nt), which regulates cancer progression via inhibiting the stability or translational efficiency of target genes [8]. Of note, circular RNAs (circRNAs) are a unique class of RNA that form a covalently closed continuous loop with no 5′-3′ polarity and no polyA tail [9]. circRNAs, which are transcribed from protein-coding genes by RNA polymerase II and synthesized by non-canonical splicing, have been shown to play critical roles in many important biological processes in human diseases [10, 11]. Previous reports have shown that circRNAs are stable, abundant and present a stage- and tissue-specific expression pattern [12–14]. Accumulating evidence has shown that circRNAs are involved in progression of several kinds of diseases [15], including nonalcoholic steatohepatitis [16], Alzheimer’s disease [17] and cancer [18–20]. However, compared with miRNAs, the mechanistic characterization and molecular functions of circRNAs in human cancers including PDAC are still poorly understood.
Previous studies have revealed that competing endogenous RNAs (ceRNAs) play important roles in post-transcriptional regulation and are involved in cancer initiation and progression. Many kinds of RNA molecules, such as pseudogenes, lncRNAs and mRNAs, can compete for the same microRNA response elements (MREs) to promote the targets of miRNAs. Recently, accumulating evidence has also revealed that circRNAs could act as ceRNAs to exercise their function at the transcriptional or post-transcriptional level [14, 21]. The first circRNA reported to act as a ceRNA was ciRS-7, which contains more than 70 conserved miR-7 target sites [21]. In 2015, Li et al. also reported that circ-ITCH harbored three miRNA binding sites (miR-7, miR-17 and miR-214) and inhibited esophageal squamous cell carcinoma (ESCC) development by suppressing the Wnt/β-catenin pathway [22]. Thus, the investigation of the interactions between the circRNA-miRNA-mRNA regulatory axis and cancer progression could provide a novel insight to explore how circRNA regulated cancer progression at a mechanistic level.
The molecular mechanisms underlying the circRNAs in PDAC remain largely unknown. To test the hypothesis that dysregulating circRNAs might be involved in the progression of PDAC, we identified differentially expressed circRNAs in PDAC by analyzing two publicly available gene expression datasets: GSE69362 [23] and GSE79634 [24]. Next, we conducted a circRNA-miRNA-mRNA regulatory axis based on in silico analysis. We performed GO and KEGG analysis to explore the potential roles of dysregulating circRNAs in PDAC. We found that DHX9 may act as a potential regulator of circRNAs formation in PDAC. These results provided useful information for exploring therapeutic candidate targets and new molecular biomarkers for pancreatic ductal adenocarcinoma.
Material and methods
Microarray data
In the present study, we focused on identifying differentially expressed circRNAs in PDAC. We screened NCBI GEO datasets (www.ncbi.nlm.nih.gov/geo/) and found two datasets involved in circRNAs in PDAC: GSE69362 and GSE79634. Furthermore, we analyzed GSE28735 to identify differentially expressed mRNAs in PDAC, which included the largest number of samples. Six pairs of human PDAC and adjacent normal tissue were contained in GSE69362. All clinical information of PDAC patients included in GSE69362 has been reported previously [25]. Meanwhile, in the GSE79634 dataset, the circRNA expression levels were measured in 20 paracancerous tissues and 20 PDAC tissues. We also analyzed GSE28735 [26, 27] (45 matching pairs of PDAC tumor and adjacent non-tumor tissues) to identify differentially expressed mRNAs. The “fold change” between the groups for each circRNA or mRNA was computed. The statistical significance of the difference may be conveniently estimated by t test. CircRNAs or mRNAs having fold changes ≥ 1.5 and p-values < 0.05 were selected as of significantly differential expression.
DHX9 protein expression in PDAC tissues was reviewed in Human Protein Atlas (http://www.proteinatlas.org/) [28].
Hierarchical clustering analysis
To generate an overview of circRNA/mRNA expression profiles between the two groups, hierarchical clustering analysis was performed based on the expression value of all targets and the most significant differentially expressed circRNA/mRNAs using Cluster and TreeView programs.
Gene ontology and pathway analysis
We conducted GO analysis to obtain meaningful annotation of genes by using the MAS system provided by CapitalBio Corporation (Molecule Annotation System, http://bioinfo.capitalbio.com/mas3/). The ontology covered domains of biological processes, cellular components and molecular functions. The enriched GO terms were presented by enrichment scores. KEGG pathway analysis was carried out to determine the involvement of differentially expressed mRNAs in different biological pathways. P < 0.05 was used as the criterion for statistical significance.
Reconstruction of the lncRNA–miRNA–mRNA network
We first used the Arraystar dataset to identify potential dysregulated circRNA-miRNA pairs. Next, StarBase and Targetscan databases were also used to identify miRNA-mRNA pairs. Finally, circRNA–miRNA–mRNA networks were constructed. In the present study, only down-regulated miRNAs and up-regulated mRNAs were integrated into the up-regulated lncRNA-mediated ceRNA network, while only up-regulated miRNAs and down-regulated mRNAs were integrated into the down-regulated lncRNA-mediated ceRNA network.
Statistical analysis
The numerical data were presented as mean ± standard deviation (SD) of at least three determinations. Statistical comparisons between groups of normalized data were performed using the t-test or Mann-Whitney U-test according to the test condition. A level of p < 0.05 was considered statistically significant with a 95% confidence level.
Results
Systematic analysis of significantly differentially expressed circRNAs in PDAC
In order to identify the differentially expressed circRNAs in PDAC, we analyzed two public datasets (GSE69362 and GSE79634) from the GEO database (Figures 1 A, B). A total of 26 pairs of human PDAC and adjacent normal tissue were analyzed in this study.
Figure 1
Systematic analysis of significantly differentially expressed circRNAs in pancreatic ductal adenocarcinoma. Heat map showing expression profiles of circRNAs in GSE69362 (A) and GSE79634 (B). The expression values are depicted in line with the color scale. The intensity increases from green to red. Each column represents one sample, and each row indicates a transcript. CircRNAs or mRNAs having fold changes ≥ 1.5 and p < 0.05 were selected as of significantly differential expression. C – Venn map showed that 169 circRNAs were upregulated and 70 circRNAs were downregulated compared with normal tissues in both GSE69362 and GSE79634 data. D, E – We conducted GO analysis to obtain meaningful annotation of genes by using the MAS system provided by CapitalBio Corporation (Molecule Annotation System, http://bioinfo.capitalbio.com/mas3/). P < 0.05 was used as the criterion for statistical significance. The link of upregulated (D) and downregulated (E) circRNAs’ parental genes among significant pathways
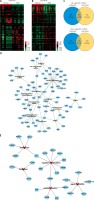
As shown in Figure 1 C, a total of 239 circRNAs were detected to be differentially expressed with fold change ≥ 1.5 and p < 0.05. Among them, 169 circRNAs were upregulated and 70 circRNAs were downregulated in PDAC compared with normal tissues in both GSE69362 and GSE79634 datasets. Clustering analysis was subsequently performed for all abnormally expressed circRNAs (Figure 1).
To explore potential functional roles of circRNAs’ parental genes, we performed GO analysis of these genes. Our results showed that up-regulated circRNAs’ parental genes were involved in regulating regulation of transcription, modification-dependent protein catabolism, interspecies interaction between organisms, protein transport and development (p < 0.05, Figure 1 D), while down-regulated circRNAs’ parental genes were involved in regulation of transcription, oxidation reduction, development, signal transduction and metabolism (p < 0.05, Figure 1 E).
Construction of the circRNA-miRNA-mRNA interaction network
To determine the function of circRNAs, interactions between circRNAs and their target miRNAs were constructed by using Arraystar data based on TargetScan [29, 30] and miRanda [31, 32] prediction. We observed that 235 miRNAs could bind to up-regulated circRNAs and 54 miRNAs could bind to down-regulated circRNAs (Figures 2 A, B).
Figure 2
Network of differentially expressed circRNAs and miRNAs. A – The up-regulated circRNA-miRNA network consists of 64 circRNAs and 235 correlated miRNAs B – The down-regulated circRNA-miRNA network consists of 12 circRNAs and 54 correlated miRNAs
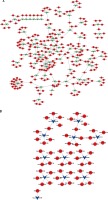
To further reveal the potential roles of circRNAs in PDAC progression, we constructed circRNA-mediated ceRNA networks. Here, we first identified differentially expressed mRNAs in PDAC using the GSE28735 dataset. A total of 765 mRNAs and 684 mRNAs were identified (Figure 3 A). Next, we used five different databases including TargetScan and Starbase [33] to predict the targets of circRNAs binding miRNAs. According to the ceRNA hypothesis, the expression of each ceRNA was positive-related. Therefore, the up-regulated or down-regulated mRNAs were selected to construct up-regulated or down-regulated circRNAs, respectively (Figures 3 B, C). A total of 51 down-regulated mRNAs were included in down-regulated circRNA-mediated ceRNA networks, and 123 up-regulated mRNAs were included in up-regulated circRNA-mediated ceRNA networks. Accordingly, the circRNA-mediated ceRNA networks were constructed using Cytoscape v3.2.1 (http://www. cytoscape.org/) software.
GO analysis of differentially expressed circRNA-mediated ceRNAs in PDAC
We performed GO analysis for differentially expressed circRNA-mediated ceRNAs in PDAC (Figure 4). According to the GO analysis, up-regulated circRNA-mediated ceRNAs were enriched in regulation of transcription, RNA splicing, signal transduction, cell cycle, cell cycle arrest, cell division, protein transport and cell-cell signaling (p < 0.05, Figure 4 A). We also found that down-regulated circRNA-mediated ceRNAs were involved in regulating transcription, metabolism, transport, protein amino acid phosphorylation, lipid metabolism, cell cycle, and cell differentiation (p < 0.05, Figure 4 B).
Figure 4
GO Analysis of differentially expressed circRNA-mediated ceRNAs in PDAC. We conducted GO analysis to obtain meaningful annotation of genes by using the MAS system provided by CapitalBio Corporation (Molecule Annotation System, http://bioinfo.capitalbio.com/mas3/). P < 0.05 was used as the criterion for statistical significance. A – The potential biological processes that were regulated by up-regulated circRNA-mediated ceRNA network. B – The potential biological processes that were regulated by down-regulated circRNA-mediated ceRNA network
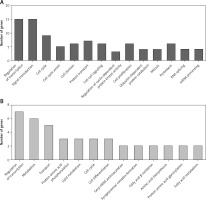
Construction of up-stream transcriptional regulatory network of differentially expressed circRNAs’ parental genes
CircRNAs are a unique class of RNA and synthesize by non-canonical splicing, suggesting that their parental genes’ expression levels may also affect circRNAs’ expression. To construct an up-stream transcriptional regulatory network for differentially expressed circRNAs’ parental genes, we download ChIP-seq data of more than 400 transcriptional factors from the ChIP database [33]. Of them, expression of about 32 TFs was dysregulated in pancreatic cancer samples compared to normal tissues, indicating that they might regulate expression of circRNAs’ parental genes. Cytoscape 3.0. was used to draw the transcriptional regulatory network (Figure 5 A). A total of 213 nodes were included in this network. Interestingly, our network contained some previously reported TFs, including TP53 [34], MYC [35], and E2F1 [36], in PDAC. We also analyzed circRNA regulator DHX9 expression between normal and PDAC samples by using GSE28735. Of note, some never reported TFs such as KLF5, ELK1, and ETS1 also played important roles and regulated more than 20 genes.
DHX9 may be a potential regulator of differentially expressed circRNAs in pancreatic ductal adenocarcinoma
Recent reports have shown that some genes (including ADAR [37], QKI [38] and FUS [39]) could regulate circRNA formation. Recently, DHX9 [40] was identified as a novel regulator of circRNAs and could suppress their formation. Thus, in this study, we also explored whether DHX9 participated in regulating differentially expressed circRNAs in PDAC. According to GSE28735 analysis, we observed that DHX9 was significantly up-regulated in PDAC with a p < 0.01 (Figure 6). To further explore potential regulation of circRNA by DHX9, we assessed the presence of DHX9 peaks in the circRNA genomic region using CLiP-seq data reported by Akhtar et al. and observed significant DHX9 peaks in hsa_circ_0006913, hsa_circ_0049392, hsa_circ_0005203, and hsa_circ_0001626 loci (Figure 7). These results suggested that DHX9 was a potential regulator of differentially expressed circRNAs in PDAC.
Figure 6
Higher expression level of DHX9 was significantly associated with shorter overall survival time. A – Relative DHX9 mRNA levels in GSE28735. DHX9 mRNA is up-regulated in PDAC with p < 0.01. B – The Kaplan-Meier curve analysis showed that higher expression level of DHX9 was significantly associated with shorter overall survival time. C – Higher protein expression of PDAC utilizing antibody staining
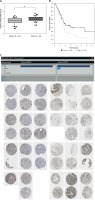
Discussion
Pancreatic ductal adenocarcinoma is one of the deadliest malignancies. However, the molecular mechanisms underlying PDAC are still not completely understood. CircRNAs are a unique class of RNAs formed by special loop splicing. More and more researchers have paid attention to circRNAs. Recently studies have shown that circRNAs were dysregulated in different kinds of tumors, including colorectal cancer, gastric cancer and bladder cancer. In PDAC, Shibin et al. also identified a series of differentially expressed circRNAs [41]. These results showed that circRNA may also act as diagnostic and prognostic markers in cancer. However, many questions remain to be answered: what, for example, are the upstream regulators that affect circRNA expression and the potential molecular functions of circRNAs in PDAC?
In this study, we analyzed two public datasets (GSE69362 and GSE79634) to identify differentially expressed circRNAs in PDAC. A total of 169 circRNAs were upregulated and 70 circRNAs were downregulated compared with normal tissues in both GSE69362 and GSE79634 data. These results were consistent with the report of Shibin et al. GO analysis of circRNAs’ parental genes showed that dysregulated circRNAs’ parental genes were involved in regulating regulation of transcription, modification-dependent protein catabolism, protein transport, oxidation reduction, development, signal transduction and metabolism.
One of most well-known functions of circRNAs is acting as miRNA sponges by binding to miRNAs to promote expression of their targets. For example, circRNA_100290 [42] was reported to function as a sponge of the miR-29 family in oral cancer. In this study, we also constructed a circRNA-miRNA-mRNA interaction network. We observed that 157 or 234 miRNAs could bind to up- or down-regulated circRNA, respectively. GO analysis for differentially expressed circRNA-mediated ceRNAs in PDAC was also performed. According to the GO analysis, up-regulated circRNA-mediated ceRNAs were enriched in RNA splicing, signal transduction, cell cycle and protein transport, while down-regulated circRNA-mediated ceRNAs were involved in regulating transcription, metabolism, transport and cell differentiation.
CircRNAs are a kind of RNAs synthesized by non-canonical splicing, suggesting that up-stream regulators could affect circRNAs’ expression via regulating their parental genes’ expression levels. Transcriptional factors were one of the most important regulators of gene expression. Here, we constructed an up-stream transcriptional regulatory network of differentially expressed circRNAs’ parental genes. We identified 32 TFs that were differentially expressed in PDAC. A total of 213 nodes were included in this network. Interestingly, our network contained some previously reported TFs in PDAC. Of note, some TFs, such as ERG, EP300, and FOXA1, regulated more than 20 genes.
Recent reports have shown that some genes could regulate circRNA formation. In 2015, authors showed the RNA Binding Protein Quaking could regulate formation of circRNAs [43]. Recently, Akhtar et al. reported that DHX9 suppressed circRNA formation. Besides these, some other proteins (including FUS, MBNL1, MBNL2, RBM20 and ADAR) were also found to be involved in circRNA regulation. In this study, we observed significant DHX9 peaks in hsa_circ_0006913, hsa_circ_0049392, hsa_circ_0005203, and hsa_circ_0001626 loci using CLiP-seq data analysis. As far as we know, this is the first study revealing the potential regulatory roles of circRNA formation in PDAC.
Several limitations of this study should be noted. First, further studies based on larger series of patients are needed to explore the differentially expressed circRNAs in PDAC. Second, additional functional investigations of these circRNAs in PDAC are still needed. Moreover, the effects of DHX9 on PDAC-related circRNA formation and PDAC progression still need to be further explored.
In conclusion, our study identified differentially expressed circRNAs in PDAC, suggesting that circRNAs may also act as biomarkers for PDAC. We constructed a circRNA-mediated ceRNA network in PDAC. GO analysis was performed to explore circRNAs’ potential roles in PDAC progression. We also constructed an up-stream transcriptional network of circRNAs’ parental genes and found that many TFs such as TP53 and MYC could regulate their expression. Finally, we observed that DHX9 acted as a potential regulator of circRNA formation in PDAC. Additional investigations on function and up-stream regulation of differentially expressed circRNAs in PDAC are still needed.