Introduction
Diabetes mellitus (DM) is a class of metabolic syndromes characterized by hyperglycemia that results from absolute or relative impairment in insulin secretion and/or insulin action, which can be induced by inherited or environmental factors [1–3]. Diabetes mellitus is one of the most common metabolic disorders in India, China, and the United States, and affected about 382 million people worldwide in 2013 [4]. Of note, an estimated 592 million DM patients will be diagnosed by 2035 [5]. Diabetic foot ulcer (DFU) is one of the most life-threatening complications of diabetes, developing in 15–25% of diabetic patients [6]. Emerging evidence indicates that DFUs impose a substantial burden on society and family because DFUs contribute to high morbidity, and hospitalization rates [7, 8]. Several studies have shown DFUs to be involved in ischemia, neuropathy, impaired immune function, and infection [9–11]. However, there is still an urgent need to develop novel biomarkers and mechanisms for DFUs.
Non-coding RNA (ncRNA) has aroused great interest and attention in biomedical research. Long non-coding RNAs (LncRNAs) are a class of endogenous RNA molecules with length of over 200 nt and lacking protein-coding potential [12]. Increasing reports have shown that lncRNAs play an important role in regulating genome integrity, X inactivation, cell cycle, cell proliferation and RNA splicing in various types of human diseases, including cancer [13–16], Hirschsprung’s disease, and nonalcoholic fatty liver disease [17]. LncRNAs can regulate protein-coding genes at the epigenetic, transcriptional, and posttranscriptional levels [18]. Several studies have revealed that lncRNAs were also associated with diabetes progression. For instance, Qiu et al. found that lncRNA MEG3 was involved in diabetes-related microvascular dysfunction [19]. Recently, Sawaya et al. reported that GAS5 participated in topical mevastatin-mediated wound healing [20]. However, the functional roles of lncRNAs remained largely unclear.
In the present study, we for the first time screened differentially expressed lncRNAs in DFU by analyzing the public dataset GSE68186. The expression patterns of four lncRNAs (FLJ30679, LINC01193, LINC00692, and LINC00641) in DFU were validated using RT-PCR. Furthermore, GO and co-expression analysis were used to explore the potential roles of dysregulating lncRNAs in DFUs. These results provided useful information for exploring therapeutic candidate targets and new molecular biomarkers for DFU.
Material and methods
Microarray data
DFU gene expression data were downloaded from the study by Ramirez et al. [21], which was referenced in the Gene Expression Omnibus (GEO) database (www.ncbi.nlm.nih.gov/geo/) under accession number GSE68186. In total, 3 DFU samples and 3 non-diabetic foot skin samples were included in this dataset. We used the arrayQuality package for quality control and the limma package to apply raw data in R software. The normalization criteria were based on quantile normalization. mRNAs having fold changes ≥ 2 and p < 0.05 were selected as of significantly differential expression.
LncRNA classification pipeline
In order to identify differentially expressed lncRNAs in GSE68186, we applied a pipeline as described by Zhang [22]. The following criteria were used to identify the unique probe sets for lncRNAs from the Affymetrix array. For the probe sets with Refseq IDs, we retained those labeled as “NR_” (NR indicates non-coding RNA in the Refseq database). Next, we filtered the probe sets obtained in step 2 by filtering out pseudogenes, rRNAs, microRNAs, tRNAs, snRNAs and snoRNAs. Finally, we obtained 2448 annotated lncRNA transcripts with corresponding Affymetrix probe IDs. LncRNAs having fold changes ≥ 2 and p < 0.05 were selected as of significantly differential expression.
Real-time reverse transcription PCR (qRT-PCR) analysis
Total RNAs were extracted using TRIzol reagent (Sigma) following the manufacturer’s instructions. Reverse transcript PCR was carried out using Prime-Script RT Master Mix (Takara, Shiga, Japan) according to the manufacturer’s instructions. Real-time qPCR was performed using AceQ qPCR SYBR Green Master Mix (Vazyme Biotech co. ltd) on a Roche LightCycler 480. The PCR primers are listed in Table I. The Ct values were normalized using β-actin as an internal control to estimate the different expression of genes. Relative mRNA expression was calculated using the 2–ΔΔCt method. Each sample was run in triplicate to ensure quantitative accuracy.
PPI network construction and module selection
The Search Tool for the Retrieval of Interacting Genes (STRING) database is an online tool designed to evaluate protein-protein interaction (PPI) information. STRING (version 9.0) covers 5 214 234 proteins from 1133 organisms. To evaluate the interactive relationships among DEGs, we mapped the DEGs to STRING, and only experimentally validated interactions with a combined score > 0.4 were selected as significant. Then, PPI networks were constructed using the Cytoscape software.
Co-expression network construction and analysis
The Pearson correlation coefficient of differentially expressed gene (DEG)-lncRNA pairs was calculated according to their expression value. We used the “cor” function of R software, which is common software. All parameters are default values. The co-expressed DEG-lncRNA pairs with the absolute value of the Pearson correlation coefficient ≥ 0.5 were selected and the co-expression network was established using cytoscape software.
Gene ontology (GO) analysis
We conducted GO analysis of genes using the STRING system (https://string-db.org/). The ontology covered domains of biological processes, cellular components and molecular functions. The enriched GO terms were presented by enrichment scores. P < 0.05 was used as the criterion for statistical significance.
Statistical analysis
All data are representative of at least three experiments of similar results performed in triplicate unless otherwise indicated. Statistical comparisons between groups of normalized data were performed using the t-test or Mann-Whitney U-test according to the test condition. A value of p < 0.05 was considered as statistically significant with a 95% confidence level.
Results
Identification and validation of differentially expressed mRNAs and lncRNAs in DFU
In this study, we analyzed the GSE68186 dataset to identify differentially expressed genes (DEGs) in DFU. In total, 811 genes were observed to be expressed differentially, including 344 upregulated genes and 467 downregulated genes. The heat map of DEGs in DFU and non-diabetic foot skin samples is shown in Figure 1 A.
Figure 1
Identification of differentially expressed mRNAs and lncRNAs in DFU. A – Hierarchical clustering analysis shows differential gene expression in the DFU by using GSE68186. DEGs analysis shows 344 up-regulated genes and 467 down-regulated genes in DFU. B – Hierarchical clustering analysis shows differential lnRNA expression in the DFU by using GSE68186. C–F – GSE68186 analysis showed that FLJ30679 (C), LINC01193 (D), LINC00692 (E), and LINC00641 (F) were up-regulated in DFU. G, K – RT-PCR analysis showed that FLJ30679 was up-regulated in DFU. H, L – RT-PCR analysis showed that LINC01193 was up-regulated in DFU. I, M – T-PCR analysis showed that LINC00692 was up-regulated in DFU. J, N – RT-PCR analysis showed that LINC00641 was up-regulated in DFU
Data are presented as the mean ± SD (n = 3). Significance was defined as p < 0.05 (*p < 0.05; **p < 0.01; ***p < 0.001); NS – not significant.
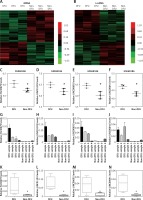
LncRNAs have been reported to play important roles in human diseases. However, the special lncRNA expression pattern in DFU had not been revealed until now. In the present study, we identified 58 up-regulated lncRNAs and 42 down-regulated lncRNAs in DFU samples compared to non-diabetic foot skin samples by applying the lncRNA classification pipeline reported by Zhang Among these lncRNAs, AC013268.1 was the most significantly up-regulated, while AL359075.1 was the most significantly down-regulated lncRNA in DFU. The heat map of differentially expressed lncRNAs in DFU is shown in Figure 1 B.
Validation of differentially expressed lncRNAs in DFU
To further confirm our analysis, four up-regulated lncRNAs (FLJ30679, LINC01193, LINC00692, and LINC00641) were randomly selected for further qRT-PCR validation. The expression levels of these lncRNAs from Agilent microarray data are shown in Figures 1 C–F. Furthermore, we performed qRT-PCR analysis to observe lncRNAs expression levels in DFU and non-DFU samples. Four lncRNAs, FLJ30679, LINC01193, LINC00692, and LINC00641, were found to be up-regulated in DFU (Figures 1 G–N). These results were consistent with our microarray data analysis.
Construction of differentially expressed protein-protein interacting network in DFU
Based on the information in the STRING database, PPI networks were constructed for DEGs between DFU and non-DFU. In the DFU up-regulated genes mediated PPI network, 113 nodes and 167 edges were included. UBC (degree = 25), HRAS (degree = 14), and SRC (degree = 11) were identified as key hub nodes (Figure 2 A). Meanwhile, 138 nodes and 222 edges were included in the DFU down-regulated genes mediated PPI network. We found that ATR (degree = 13), RAD52 (degree = 13), MRPL19 (degree = 11), RAD50 (degree = 11) and RPS3 (degree = 11) were the key hub nodes with the highest connectivity degree (Figure 2 B).
Co-expression network analysis
In order to evaluate the potential roles of differentially expressed lncRNAs in DFU progression, we constructed up- and down-regulated lncRNA-mRNA co-expressing networks. The co-expressed lncRNA-mRNA pairs with the absolute value of Pearson’s correlation coefficient ≥ 0.9 were selected for network construction. We found that the up-regulated lncRNA mediated co-expression network contained 58 lncRNAs and 688 DEGs (Figure 3) and the down-regulated lncRNA mediated co-expression network contained 42 lncRNAs and 700 DEGs (Figure 4). Here, we identified down-regulated LINC00638 (co-expressing with 83 DEGs), AL359075.1 (co-expressing with 82 DEGs), AC013460.1 (co-expressing with 79 DEGs), LINC00535 (co-expressing with 78 DEGs), and up-regulated FAM170B-AS1 (co-expressing with 52 DEGs), MAN1B1-AS1 (co-expressing with 51 DEGs), AC096553.1 (co-expressing with 47 DEGs), and REG1CP (co-expressing with 45 DEGs) as key regulators in DFU.
Functional annotation of differentially expressed lncRNAs
We next performed bioinformatics analysis for differentially expressed lncRNAs in DFU by using ClueGO, which is a Cytoscape plug-in to decipher functionally grouped gene ontology and pathway annotation networks. Co-expressing DEGs of up- and down-regulated lncRNAs were classified according to the GO term. As shown in Figure 5, up-regulated lncRNAs were associated with regulation of the ERK1 and ERK2 cascade, the stress-activated protein kinase signaling cascade, the secondary alcohol biosynthetic process, neurotrophin production and cornification (Figure 5 A). We also found that down-regulated lncRNAs were associated with cilium assembly and organization, centrosome duplication and DNA repair (Figure 5 B).
Discussion
Long non-coding RNAs (LncRNAs) are a class of endogenous non-coding RNA molecules with length of over 200 nt [12]. Previous studies have shown that lncRNAs play important roles in regulating a series of biological processes in human diseases, including diabetes mellitus [17]. Diabetes mellitus is one of the most common metabolic disorders. Recent reports indicated that dysregulation of lncRNAs was associated with DM progression. For example, lncRNA MALAT1 was found to be overexpressed [23] and circulating lncRNA GAS5 was down-regulated in DM compared to the normal control [24]. Furthermore, Lin et al. [25] constructed a competitive endogenous RNA network in type 2 diabetes mellitus which contained 351 nodes including 98 mRNAs, 86 microRNAs and 167 lncRNAs. These studies demonstrated the potential roles of lncRNAs in DM. Diabetic foot ulcer (DFU) is one of the most life-threatening complications of diabetes, developing in 15–25% of diabetic patients [11]. However, very few studies except that of Sawaya et al. have focused on exploring the special regulatory roles and expression pattern of lncRNAs in DFUs.
In the present study, we identified 58 up-regulated lncRNAs and 42 down-regulated lncRNAs in DFU samples compared to non-diabetic foot skin samples by analyzing the GSE68186 dataset. Among these lncRNAs, AC013268.1 was the most significantly up-regulated, while AL359075.1 was the most significantly down-regulated lncRNA in DFU. To further confirm our analysis, 4 lncRNAs (FLJ30679, LINC01193, LINC00692, and LINC00641) were randomly selected for further qRT-PCR validation. Consistent with our microarray analysis, we observed that FLJ30679, LINC01193, LINC00692, and LINC00641 were up-regulated in DFU. As far as we know, this is the first study to identify differentially expressed lncRNAs globally. These results suggest the potential prognostic value of lncRNAs in DFU.
LncRNAs can regulate genome integrity, X inactivation, cell cycle, cell proliferation and RNA splicing in various types of human diseases by affecting targets at the epigenetic, transcriptional, and posttranscriptional levels [26]. However, the molecular functions and mechanisms of lncRNAs in DFU have remained largely unclear. In this study, we constructed lncRNAs co-expression networks in DFU. We found that the down-regulated lncRNA mediated co-expression network contained 42 lncRNAs and 700 DEGs and the up-regulated lncRNA mediated co-expression network contained 58 lncRNAs and 688 DEGs. Six lncRNA (LINC00638, AL359075.1, AC013460.1, LINC00535, FAM170B-AS1, and MAN1B1-AS1) were identified as key regulators in DFU progression by regulating more than 50 DEGs. Bioinformatics analysis revealed that up-regulated lncRNAs were associated with regulation of the ERK1 and ERK2 cascade, the stress-activated protein kinase signaling cascade, the secondary alcohol biosynthetic process, neurotrophin production and cornification. We also found that down-regulated lncRNAs were associated with cilium assembly and organization, centrosome duplication and DNA repair.
Of note, several proteins were also identified as key regulators in DFU. A total of 344 upregulated genes and 467 downregulated genes were found in DFU. Furthermore, PPI networks were for the first time constructed, and up-regulated UBC (degree = 25) and HRAS (degree = 14), and down-regulated ATR (degree = 13) and RAD52 (degree = 13), were identified as key proteins.
Several limitations of this study should be noted. We detected candidate lncRNAs expression levels in 3 DFU samples and 3 non-diabetic foot skin samples. The sample size is very small. In the further study, we should evaluate the correlation between expression levels of key lncRNAs and DFU progression in a large sample size, which will be useful for exploring whether lncRNAs could serve as DFU biomarkers. Moreover, the functional roles of key lncRNAs and proteins in DFU still need to be further validated. Loss/gain of function assays should be performed in the further study.
In conclusion, we identified 811 mRNAs and 100 lncRNAs that were significantly differentially expressed in DFU. To reveal the novel mechanisms underlying DFU progression, we for the first time constructed differentially expressed mRNA mediated PPI networks and lncRNA co-expression networks. Although several limitations should be noted in this study, these results suggest that specific lncRNAs could be valuable for exploring therapeutic candidate targets and new molecular biomarkers for DFU.