Introduction
Pancreatic adenocarcinoma (PAAD) is a leading cause of cancer-related mortality worldwide, predominantly affecting middle-aged and older individuals [1]. Pancreatic ductal adenocarcinoma (PDAC) represents the most common subtype of PAAD, accounting for 85–95% of solid pancreatic tumors [2]. PAAD is a highly aggressive malignancy, often presenting with non-specific symptoms such as upper abdominal discomfort, pain, weight loss, anorexia, nausea, vomiting, abdominal distention, and altered bowel habits [3, 4]. The etiology of PAAD is multifactorial, encompassing smoking, alcohol consumption, high-fat diets, environmental pollution, and genetic predispositions [5, 6]. Despite advances in treatment, the poor prognosis of PAAD and inter-individual heterogeneity necessitate further research to enhance therapeutic outcomes.
Precision oncology represents an individualized diagnostic and therapeutic approach tailored to the unique molecular and biopathological characteristics of patients, offering promising potential for advancing our understanding of tumor biology and improving prognosis [7, 8]. Multi-omics is a comprehensive biological research approach that involves the simultaneous analysis of multiple biological molecular layers, such as genomics, transcriptomics, proteomics, metabolomics, and more, to gain a holistic understanding of the structure, function, and regulatory mechanisms of biological systems. It has a wide range of applications in various aspects, such as cancer classification and identification of molecular subtypes: by analyzing data at multiple levels, such as genome, transcriptome, proteome, etc., it is possible to classify different types of cancers in a finer way and identify different molecular subtypes, which can help to formulate a more targeted therapeutic strategy. Discovery of targeted therapies: Multi-omics can reveal abnormal gene expression and protein abnormalities in cancer cells, which can lead to the discovery of new therapeutic targets and accelerate drug development. Response prediction: By analyzing transcriptomic and metabolomic data, it is possible to predict the response of patients to specific therapeutic regimens, helping doctors to choose the most appropriate treatment. Biomarker discovery: Multi-omics can identify potential biomarkers in serum, urine and other samples for early diagnosis and disease monitoring. In the context of PAAD precision medicine, researchers often employ multi-omics to identify novel therapeutic targets, establish biospecimen repositories for preclinical drug research, and conduct prospective clinical trials. Qian et al. reviewed the trajectory of precision oncology for PDAC, highlighting three tumor suppressors associated with PDAC: TP53, CDKN2A, and SMAD4. Notably, SMAD4 mutation status may serve as a molecular typing tool in PDAC precision oncology [9]. Wang et al. reported the overexpression of PLAU in PAAD and its correlation with poor patient outcomes [10]. Furthermore, other studies identified the role of SMARCD3 as an epigenetic regulator in PAAD, influencing drug resistance and prognosis [11]. Although recent investigations have yielded progress in PAAD research, additional studies are required to improve patient survival and prognosis.
Given its aggressive nature and poor prognosis, PAAD demands continued investigation [12]. In this study, we employed bioinformatics techniques to analyze PAAD-related datasets, aiming to identify a hub gene associated with PAAD prognosis. Through this analysis, we discovered CENPN (centromere protein N) as the hub gene. As one of two pillars for kinetochore assembly at the centromere, CENPN recognizes the CENP-A nucleosome through its amino-terminal region, while forming a heterodimer with CENP-L through its carboxy-terminal region to recruit other CCAN (constitutive centromere-associated network) proteins [13]. Recently, the relevance of CENPN to the occurrence and progression of different cancers has been proposed. In oral squamous cell carcinoma, CENPN knockdown arrests the cell cycle in the G1 phase, which leads to suppression of cellular proliferation [14]. CENPN was shown to promote hepatocellular carcinoma cell proliferation and CENPN deficiency was shown to increase radiotherapy-induced DNA damage [15]. Cell experiments showed that CENPN deficiency impaired cell proliferation, migration and invasion ability and increased glioma apoptosis. [16]. We subsequently explored the involvement of CENPN in PAAD through clinical parameters, drug sensitivity assays, and in vitro cellular experiments. These findings offer valuable insights into the underlying mechanisms of PAAD and suggest potential targets for the development of novel therapeutic strategies.
Material and methods
Analysis of microarray data
Three microarray datasets, GSE16515, GSE32676, and GSE125158, were downloaded from the Gene Expression Omnibus (GEO) database (https://www.ncbi.nlm.nih.gov/geo/). GSE16515 included 32 tumor samples and 16 normal samples, GSE32676 25 PDAC samples and 7 non-malignant pancreas samples, and GSE125158 17 pancreatic cancer patient data samples and 7 normal samples.
Identification of DEGs
The GEO2R tool in the GEO database was applied to screen differentially expressed genes (DEGs) from the above three microarray datasets (GSE16515, GSE32676, GSE125158), and corrected by adjusting the p-value and calculating the Benjamini-Hochberg false discovery rate. Among them, the screening standard for up-regulated DEGs was fold change (FC) > 1.5, and the screening standard for down-regulated DEGs was FC < 0.67, and both met p < 0.05. After that, the overlapping DEGs in the three microarray datasets were respectively visualized using Venn diagrams for subsequent analysis.
PPI network analysis of DEGs
The protein-protein interaction (PPI) network analysis of up-regulated DEGs and down-regulated overlapping DEGs was performed by the Search Tool for the Retrieval of Interacting Genes (STRING) online tool (https://string-db.org/), and visualized by Cytoscape software.
Enrichment analysis of DEGs
Then, the “Cluster Profiler” package of R software was employed to analyze the Gene Ontology (GO) function and Kyoto Encyclopedia of Genes and Genomes (KEGG) pathway enrichment of the genes in the PPI network.
Construction of risk model by LASSO Cox regression analyses
On 99 genes in the PPI network, Least Absolute Shrinkage and Selection Operator (LASSO) Cox regression analysis was used to identify the signature prognostic genes and create a risk score model. The median cutoff value was used to categorize PAAD patients into a high-risk and a low-risk group in The Cancer Genome Atlas (TCGA) database. Progression-free survival (PFS) was compared between the two groups using a Kaplan-Meier (KM) analysis and a log-rank test, and the corresponding p-value, hazard ratio (HR), and 95% confidence interval (95% CI) were computed. Finally, “timeROC” was applied to draw the receiver operating characteristic (ROC) curve of the risk model, and assess the prediction accuracy by calculating the area under the curve (AUC) value of the model in 1, 3, and 5 years.
cBioPortal website analysis
cBioPortal (http://www.cbioportal.org/) is an open web platform for cancer data analysis developed by the TCGA database. In the current study, we performed mutation type and mutation frequency analysis in PAAD for 17 genes included in the risk model. The KM survival curve was then used to analyze the disease-free survival rate of 17 genes, and the log-rank test was applied to calculate the p-value.
Survival and expression analysis of genes in public databases
To screen out the genes associated with PAAD prognosis from the 17 genes in the risk model, we performed survival analysis and expression validation on these genes through public databases. Firstly, the effect of differential expression of these genes on the overall survival (OS) probability of PAAD samples was tested in the Gene Set Cancer Analysis (GSCA, http://bioinfo.life.hust.edu.cn/GSCA/#/), and the p-value was calculated by the log-rank test. Next, the Gene Expression Profiling Interactive Analysis (GEPIA) database (http://gepia.cancer-pku.cn/), which tracks gene expression, was used to evaluate the expression of these genes in PAAD tumor tissues. Through the above analysis method, the hub gene of this study was determined.
Clinical parameters and drug susceptibility analysis of CENPN
After determining the hub gene of this study, the UALCAN database (http://ualcan.path.uab.edu) was employed to detect CENPN levels in different clinical parameters of PAAD, including patient’s gender, patient’s drinking habits and TP53 mutation status. Subsequently, the PAAD samples of high and low CENPN expression were downloaded in TCGA. According to the Genomics of Drug Sensitivity in Cancer (GDSC) database, the IC50 treatment responses of CENPN high and low expression samples to three PAAD therapeutic drugs (docetaxel, paclitaxel, sunitinib) were predicted. The difference between the two groups was tested by the Wilcoxon method.
Gene set enrichment analysis (GSEA)
In order to explore the specific pathway of CENPN in PAAD, we performed GSEA on the gene, analyzed its main enrichment pathways in KEGG, and selected a key pathway for subsequent in vitro cell experiments. When p < 0.05, the obtained enrichment results are statistically significant.
Cell culture and transfection
Normal human pancreatic ductal epithelial cell (HPDE) and 4 PAAD cell lines (BxPC-3, PANC-1, AsPC-1, CFPAC-1) from the Chinese Academy of Sciences (Shanghai, China) were kept under specific conditions (5% CO2, 37°C) in DEME containing 10% FBS. Shanghai Genechem provided small interfering RNA (siRNA) and lentiviral vector (pcDNA3.1-CENPN) of CENPN, as well as lentiviral vector of MDM2 (pcDNA3.1-MDM2). Then, the PAAD cells were transfected with Lipofectamine 3000 according to the instructions.
Quantitative real-time PCR (qRT-PCR)
Trizol reagent (Invitrogen) was used to extract total RNA from cultured cells, and the purity and concentration of RNA were determined by spectrophotometry. Using a PrimeScript RT reagent kit (Takara, Dalian, China), 500 ng of RNA was reverse transcribed into cDNA. SYBR Green Premix EX Taq (Takara) and specific primers were used for the PCR reaction. qRT-PCR was conducted on the Bio-Rad CFX96 PCR system, with GAPDH as the internal control. The primers used in the study are as follows: CENPN forward, 5′-CTGAGGCACAGCTGAAAACC-3′ and reverse, 5′-AGGACAGCTGCTTGCTTTATG-3′; GAPDH forward, 5′-GAGTCAACGGATTTGGTCGT-3′ and reverse, 5′-GACAAGCTTCCCGTTCTCAG-3′. Finally, the relative mRNA expression was calculated by 2−ΔΔCt methods.
Western blot (WB) assay
From PAAD cells, total protein was extracted. The concentration of the extracted protein was measured using the BCA Protein Concentration Kit. Then, proteins were transferred to PVDF membranes after being separated on SDS-PAGE gels. The membranes were then incubated with primary antibodies against CENPN (Abcam), MDM2 (Abcam), p53 (Abcam), p21 (Abcam), or actin (Cell Signaling Technology) overnight at 4°C, followed by an hour-long incubation with secondary antibodies that were horseradish peroxidase-conjugated (Cell Signaling Technology). An electrochemiluminescence (ECL) detection system (Bio-Rad) was used to observe the protein bands, and ImageJ was used to quantify them.
Cell proliferation assay
At a density of 3 × 103, the transfected cells were infused onto a 96-well plate, and 10 μl of Cell Counting Kit-8 (CCK-8) reagent was added dropwise to the well plate. Subsequently, the optical density (OD) value of the cells at 450 nm was detected at 0, 24, 48, 72 and 96 h to evaluate the cell proliferation activity.
Cell migration and invasion assay
Transwell technology was used to measure the migration and invasion activity of transfected PAAD cells. The transfected cells were first placed in the upper chamber containing Matrigel (Matrigel is available for invasion assays and not required for migration assays). After a period of incubation, excess cells were wiped off with cotton swabs, methanol was used to fix migratory and invasive cells, and they were stained with DAPI. Finally, the number of migrating and invading cells was observed using a microscope.
Co-immunoprecipitation
Co-immunoprecipitation (Co-IP) was conducted to detect the interaction between MDM2 and p53 in CENPN-knockdown pancreatic cancer cells. Briefly, using IP buffer enhanced with protease inhibitors, cells were lysed. The lysates were first treated with an anti-MDM2 antibody for a whole night at 4°C, then with protein A/G-agarose beads for 2 h at the same temperature. Next, the immunoprecipitates were cleaned, eluted, and subjected to WB analysis.
Flow cytometry
Using flow cytometry and an Annexin V-FITC/PI apoptosis detection kit, apoptosis was quantified. Cells were transfected with siRNA or overexpression plasmids and treated with MDM2 overexpression or the p53 inhibitor PFTα. After 48 h, cells were taken from the culture, labeled with PI and Annexin V-FITC, and then examined by flow cytometry.
Statistical analysis
Each experiment was carried out at least three times, and the outcomes were then evaluated with the aid of the statistical program SPSS 22.0 and presented as mean and standard deviation (SD).
The significance of differences between the means of the different groups was examined using Student’s t-test. It was deemed significant at p < 0.05 (Supplementary Table SI).
Results
Determination of 161 overlapping DEGs
We screened 3213 DEGs, 2390 DEGs, and 6556 DEGs from the GSE16515, GSE32676, and GSE125158 datasets, respectively, where red was up-regulation and purple was down-regulation, as shown in Figures 1 A–C. Next, we found 161 overlapping DEGs from 3 microarray datasets, including 92 up-regulated and 69 down-regulated DEGs (Figures 1 D, E).
Figure 1
Screening of overlapping DEGs in three microarray datasets. A – Volcano map of DEGs in the GSE16515 dataset; the purple scatter points on the left are 865 down-regulated DEGs, and the red scatter points on the right are 2348 up-regulated DEGs. B – Volcano map of DEGs in the GSE32676 dataset; the purple scatter points on the left are 970 down-regulated DEGs, and the red scatter points on the right are 1420 up-regulated DEGs. C – Volcano map of DEGs in the GSE125158 dataset; the purple scatter points on the left are 5517 down-regulated DEGs, and the red scatter points on the right are 1039 up-regulated DEGs. D – Venn diagram showing 92 overlapping up-regulated DEGs in the 3 datasets. E – Venn diagram showing 69 overlapping down-regulated DEGs in the 3 datasets
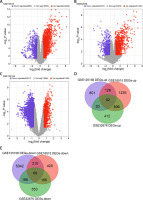
Enrichment analysis of genes in PPI network
The PPI network of 161 overlapping DEGs contained 99 nodes and 430 edges (Figure 2 A). The “Cluster Profiler” package performed GO and KEGG analysis on 99 genes (Figures 2 B–E). In GO terms, the gene enrichment items included Kinetochore organization, Mitotic sister chromatid segregation, Centromere complex assembly, Mitotic spindle, Chromosome, O-acyltransferase activity, etc. In addition, the top 10 KEGG pathways enriched by 99 genes also included p53 signaling pathway, Thyroid cancer, IL-17 signaling pathway, Glycerophospholipid metabolism, PPAR signaling pathway, etc.
Figure 2
Integrated analysis of overlapping DEGs, risk model construction, and genetic mutations in PAAD. A – The PPI network established by the STRING online tool and Cytoscape software; nodes represent genes, and edges represent the interconnection between genes. B–E – Bubble diagram: the top 10 enrichment items of the 99 genes in the PPI network in BP, CC, MF, and KEGG, where the larger the bubble is, the greater is the number of enriched genes. F – LASSO Cox regression analysis of 99 genes in the PPI network; different colored lines represent different genes. G – The horizontal axis is log(λ), the vertical axis is the partial likelihood deviation, the dashed line on the left is the minimum value of log(λ), and the corresponding number is the signature gene coefficient. H – Risk model for selected samples. The upper figure is a scatter plot of low-risk samples and highrisk samples, the middle scatter plot shows the different survival status of patients, and the lower figure is a heat map of the expression distribution of 17 signature genes in two groups of risk samples. I – KM survival curves of the high-risk group and the low-risk group; the horizontal axis represents the survival time in years, and the vertical axis represents the progression-free survival probability. J – For the ROC curve of the risk model in 1, 3, and 5 years, the horizontal axis is represented by the false positive fraction, and the vertical axis is represented by the true positive fraction. The higher the AUC value is, the higher is the prediction accuracy. K – cBioportal analyzes the percentage of genetic alterations in 17 genes in different PAAD subtypes. L – Percentage of alterations in 17 genes in PAAD; the left column represents the gene name, the percentage number represents the mutation frequency, the bars arranged horizontally represent the samples in the data set, and the different colors represent different mutation types. M – Analysis of the disease-free survival rate of 17 genes in the cBioPortal database; the red line represents the Altered group, and the blue line represents the Unaltered group. p < 0.05 was considered statistically significant
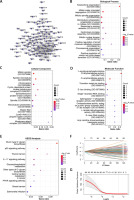
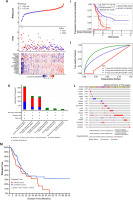
Prognostic value of 17 genes in the risk model
The 99 genes in the PPI network were subjected to LASSO Cox regression analysis, and the individual variable coefficients near to zero steadily grew as decreased. At the minimum value of λ (0.0622), we selected 17 genes as signature prognostic genes (Figures 2 F, G). Next, based on the median of 17 genes’ expression in PAAD, we built and analyzed the risk model for the high (n = 89) and low-risk group (n = 90) of PAAD. In the high-risk group, the survival time of patients decreased and the number of deaths increased, and 17 genes also had significantly different expression distributions between the two risk groups (Figure 2 H). Then KM survival analysis was conducted on the two groups, in which the PFS probability in the high-risk group was poor, and the HR value of the high-risk sample corresponding to the risk sample was 3.17 (HR > 1), indicating that the model was a hazard model (Figure 2 I). Finally, in the ROC curve analysis results of the risk model, the AUC value of the model at 5 years was 0.947, which was higher than the AUC values at 1 and 3 years, indicating that the model has the highest prediction accuracy at 5 years (Figure 2 J).
Genetic alteration analysis of 17 genes in PAAD
Through the cBioPortal website and TCGA database, we carried out mutation analysis on 17 genes. As shown in Figure 2 K, mutation was the only type of alteration observed in all subtypes of PAAD. Among the 17 genes, MYC had the highest substitution rate of 6%. In terms of genetic variation types, amplification, deep deletion, and missense mutation (unknown significance) were also the main types of genetic variation of other genes (Figure 2 L). Subsequently, we found that the disease-free survival rate of these genes in the Altered group was significantly worse than in the Unaltered group (Figure 2 M).
Prognostic significance of differential expression of 9 genes in PAAD
Survival and expression analyses of the 17 genes in the risk model were performed through the GSCA website and the GEPIA database, respectively (Figures 3 A–R). Among the 17 genes, the expression of 9 genes had a significant association with PAAD patient survival (log-rank p < 0.05). High expression of CENPN, CCNB2 CEP55, TNFSF, HIST1H2AC, LPCAT2, and SAMD was associated with poorer OS probability in PAAD patients. These seven genes were found to be highly expressed in the PAAD tumor samples, according to the findings of the GEPIA analysis. The other two genes, EPM2A and C25A45, on the other hand, had low expression in PAAD tumor samples and were associated with better survival in PAAD patients.
Figure 3
Survival and expression analysis of genes in GSCA and GEPIA databases. A, C, E, G, I, K, M, O, Q – The influence of differential expression of 9 genes (CENPN, CCNB2, CEP55, TNFSF10, EPM2A, HIST1H2AC, LPCAT2, SAMD9, SLC25A45) on the OS probability of PAAD patients; the red line indicates high expression, the purple line indicates low expression, the horizontal axis is the survival time, the vertical axis is the probability of OS, and the corresponding P value is calculated by the log-rank test. B, D, F, H, J, L, N, P, R – Boxplot of the expression verification of 9 genes (CENPN, CCNB2, CEP55, TNFSF10, EPM2A, HIST1H2AC, LPCAT2, SAMD9, SLC25A45) in PAAD tumor samples; the red color indicates the tumor samples, and the purple box indicates the normal samples. *p < 0.05
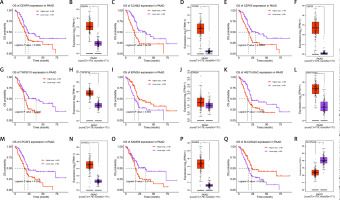
Expression and drug resistance analysis of CENPN in PAAD
The UALCAN database evaluated the relation between CENPN and clinical parameters of PAAD. In Figures 4 A–C, the expression of CENPN was not significantly different in different groups according to patient’s gender or patient’s drinking habits. But in TP53 mutation status, the expression of CENPN in TP53-nonmutant was slightly lower than that in TP53-mutant. In the GDSC database, the Wilcoxon test showed that the IC50 values of gemcitabine, docetaxel, paclitaxel, and sunitinib were lower in the high group but higher in the low group (Figures 4 D–G). This indicated that these 4 drugs had higher sensitivity in samples with high expression of CENPN.
Figure 4
Clinical feature analysis of CENPN by public databases. A – Expression of CENPN in PAAD based on patient’s gender was detected in UALCAN database. B – Expression of CENPN in PAAD based on patient’s drinking habits was detected in UALCAN database. C – Expression of CENPN in PAAD based on TP53 mutation status was detected in UALCAN database. D – GDSC database detected distribution of gemcitabine IC50 scores in CENPN high expression group and CENPN low expression group, ****p < 0.0001. E – GDSC database detected distribution of docetaxel IC50 scores in CENPN high expression group and CENPN low expression group, *p < 0.05. F – GDSC database detected distribution of paclitaxel IC50 scores in CENPN high expression group and CENPN low expression group, ****P < 0.0001. G – GDSC database detected distribution of sunitinib IC50 scores in CENPN high expression group and CENPN low expression group, *p < 0.05
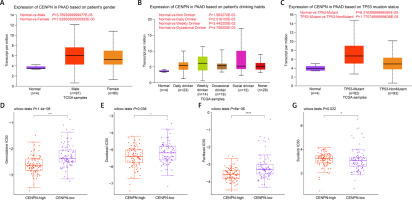
The effect of CENPN on PAAD cells in vitro
Next, qRT-PCR technology detected that CENPN had higher expression in PAAD cell lines than in normal pancreatic ductal epithelial cells, particularly in PANC-1 and BxPC-3 cells (Figure 5 A and Supplementary Table SII). Similarly, WB also detected that the protein level of CENPN was also higher in PANC-1 and BxPC-3 cells (Figure 5 B). To investigate the effects of CENPN expression on PAAD cell proliferation, knockdown and overexpression experiments were performed, and WB confirmed the efficiency (Figures 5 C, D). In the cell proliferation assay, CCK-8 technology detected that the low expression of CENPN suppressed cell growth, while the overexpression promoted it (Figures 5 E–H). In cell migration and invasion experiments using Transwell technology to detect the number of migrating and invading PAAD cells, si-CENPN decreased PAAD cell migration and invasion (Figures 6 A, B), while over-CENPN promoted it (Figures 6 C, D).
Figure 5
Effect of CENPN knockdown on PAAD cells. A – mRNA levels of CENPN in normal pancreatic ductal epithelial cell lines and PAAD cell lines were detected by qRT-PCR. B – Protein levels of CENPN in normal pancreatic ductal epithelial cell lines and PAAD cell lines were detected by WB. C – Knockdown efficiency of CENPN in PAAD cells was detected by WB. D – Overexpression efficiency of CENPN in PAAD cells was detected by WB. E, F – Regulation of si-CENPN on PAAD cell proliferation was detected by CCK-8. G, H – Regulation of over-CENPN on PAAD cell proliferation was detected by CCK-8. *p < 0.05, **p < 0.01
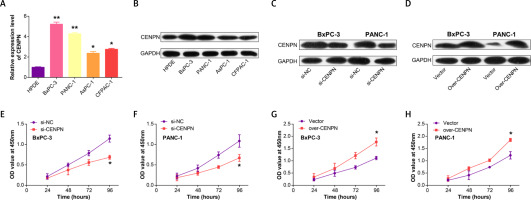
Figure 6
CENPN promotes PAAD migration and invasion in vitro. A, B – The effect of si-CENPN on PAAD cell migration was determined by Transwell assay. The upper panels are representative images of cell migration and invasion after staining, and the lower panels are the quantification of migrated/invaded cells. C, D – The effect of over-CENPN on PAAD cell migration was determined by Transwell assay. The upper panels are representative images of cell migration and invasion after staining, and the lower panels are the quantification of migrated/invaded cells. *p < 0.05, **p < 0.01
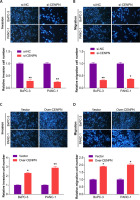
CENPN inhibits PAAD cell apoptosis by regulating MDM2-mediated p53 pathway
After the GSEA-KEGG enrichment analysis of CENPN, 7 pathways were obtained, namely Nucleotide excision repair, Cell cycle, Oocyte meiosis, p53 signaling pathway, Mismatch repair, DNA replication, and Homologous recombination (Figure 7 A). Among them, the p53 signaling pathway is reported to be related to the pathogenesis of PAAD, affecting the proliferation and senescence of PAAD cells. MDM2 is an important negative regulatory protein that plays a key role in cells [17]. Previous studies have shown that this protein can combine with p53 to regulate the stability and activity of p53, thereby affecting the growth of cells [18, 19]. Therefore, we speculate that CENPN may affect the expression level of p53 through the interaction with MDM2 protein. WB technology detected that after knocking down the level of CENPN in PANC-1 and BxPC-3 cells, the protein level of MDM2 was down-regulated, while the protein levels of p53 protein as well as the downstream target p21 protein were up-regulated (Figure 7 B). From the results of Co-IP assay, knockdown of CENPN decreased the interaction between MDM2 protein and p53 protein compared with the control group (Figure 7 C). Subsequently, flow cytometry showed that down-regulating CENPN can promote cell apoptosis, while PFTα (p53 inhibitor) and overexpressed MDM2 protein can weaken the promoting effect of si-CENPN on cell apoptosis (Figures 7 D, E).
Figure 7
Mechanism of CENPN in PAAD through MDM2-mediated P53 signaling pathway. A – GSEA-KEGG enrichment analysis of CENPN, showing the top seven pathways. B – Protein expression levels of MDM2, p53 and its downstream target p21 were detected by WB after CENPN knockdown in BxpPC-3/PANC-1 cells. C – Co-IP analysis showing the interaction between MDM2 and p53 in BxpPC-3/ PANC-1 cells after knockdown of CENPN, and detected by WB using the indicated antibodies. D – Flow cytometric analysis of apoptosis levels in BxpPC-3/PANC-1 cells after knockdown of CENPN and overexpression of MDM2 or treatment with P53 inhibitors (PFTα)
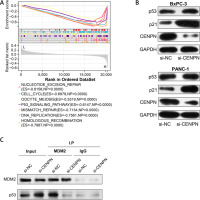
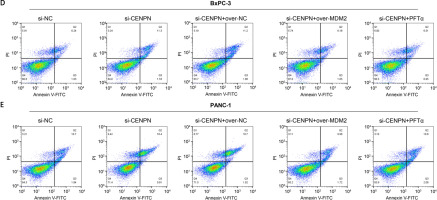
Discussion
Pancreatic adenocarcinoma (PAAD) is currently one of the most aggressive and malignant tumors [20]. Pancreatic adenocarcinoma (PAAD) is characterized by high incidence of malignancy, a low resection rate, a high recurrence rate and poor prognosis. Also, as it does not cause immediate symptoms, it is difficult to detect early [21, 22]. Diagnosing pancreatic cancer is challenging, with most cases occurring in advanced or metastatic disease [23, 24]. Ductal adenocarcinomas originating from the ductal epithelium represent the predominant histological subtype of PAAD, which is associated with a high degree of malignancy and a poor prognosis [25, 26]. The incidence of PAAD has been steadily increasing in recent years, with most patients presenting symptoms of distant metastasis [27, 28]. Despite the use of surgery, chemotherapy and other therapeutic modalities, effective treatment options for PAAD are limited and often associated with varying degrees of tolerance [29] as well as high recurrence rates and low long-term survival [24, 30]. To overcome these challenges, researchers have shifted their focus towards investigating the biological mechanisms underlying PAAD, with the aim of identifying novel therapeutic targets through bioinformatics approaches.
In our study, DEGs in GSE16515, GSE32676, GSE125158 microarray datasets were first screened, and their overlapping DEGs were identified. Then, we constructed the PPI network for the overlapping DEGs, and obtained 99 interactive genes, whose enrichment items in GO and KEGG included Kinetochore organization, Centromere complex assembly, Mitotic spindle, Chromosome, O-acyltransferase activity, IL-17 signaling pathway, Glycerophospholipid metabolism, p53 signaling pathway, etc. Among them, Hamada et al. mentioned in their research that mitotic spindle assembly might be a common feature of lung cancer and PAAD [31]. Cao et al. found that the gene could affect the cell growth process of PAAD by inhibiting p53 and activating the mTORC1 signaling pathway when exploring the mechanism of LEMD1 in PAAD [32]. Zhang et al. observed that loss of IL17/IL17R signaling led to growth inhibition of PAAD [33]. Some studies have found that IL-17A factor was related to the development of PAAD [34, 35]. Genetic variation in some PPAR pathway genes may also increase susceptibility to PAAD. Lai et al. also confirmed that PAAD was related to some tumor signaling pathways, including the PPAR signaling pathway [36]. Therefore, we speculate that these genes may be involved in the pathogenesis of PAAD through the above-mentioned enrichment items.
To identify genes associated with PAAD prognosis, a LASSO Cox analysis was performed on 99 candidate genes, resulting in the identification of 17 signature genes through risk model analysis. Subsequently, the cBioPortal website was utilized to examine mutations and survival of these 17 genes, revealing that amplification was the main mutation type and that disease-free survival rates were low in the Altered group. The GSCA database was then used to evaluate the impact of differential expression of these genes on PAAD survival, indicating that high expression of 7 genes (CENPN, CCNB2, CEP55, TNFSF, HIST1H2AC, LPCAT2, SAMD9) was associated with poor prognosis, while EPM2A and C25A45 were associated with higher probability of overall survival in patients. Among these 9 genes, only the relationship between CENPN and PAAD has not been previously reported. Therefore, after a comprehensive bioinformatics analysis of 161 concordant differentially expressed genes (DEGs) in three microarray datasets, CENPN emerged as the central gene of the study.
CENPN (centromere protein N) has been reported to be related to the pathogenesis of various tumors and can be used as a potential therapeutic target [37, 38]. Wu et al. proposed that CENPN level not only had a close association with the infiltration of immune cells in glioma, but also affected cell proliferation, invasion and migration capabilities [16]. Through bioinformatics analysis, Wang et al. found that CENPN could be a prognostic biomarker for breast cancer [39]. CENPN has also been confirmed to be overexpressed in lung adenocarcinoma, and can target the PI3K/AKT pathway to affect the tumorigenesis of tumors [40]. To study the specific biological function of CENPN in PAAD, we analyzed the clinical parameters and drug resistance of the gene. The UALCAN database showed that CENPN level varied significantly among TP53 mutation status groups. Furthermore, in the context of treating pancreatic adenocarcinoma (PAAD), it has been observed that in samples with high CENPN expression, there is increased sensitivity to four PAAD therapies: gemcitabine, docetaxel, paclitaxel, and sunitinib. This suggests a correlation between the overexpression of CENPN in PAAD and the heightened responsiveness of patients to these four therapeutic approaches. Based on these results, we speculated that the expression of CENPN may affect the effectiveness of clinical drug treatment of PAAD.
We further investigated the role of CENPN in PAAD by examining its expression in PAAD cell lines. We found that CENPN had high expression in these cells, suggesting that this gene could serve as a proto-oncogene of PAAD. Knockdown of CENPN resulted in suppressed cell growth, migration, and invasion of PAAD cells, whereas upregulation of CENPN significantly promoted cell proliferation, invasion, and migration. As the p53 signaling pathway is known to be related to the pathogenesis of PAAD [12, 41], we analyzed the possible effects of CENPN in combination with this pathway. Western blot analysis showed that downregulation of CENPN in PAAD cells upregulated the expression of MDM2 and downregulated the expression of p53 and its downstream target p21, indicating that CENPN may play a role in regulating the P53 pathway in PAAD. MDM2 is an E3 ubiquitin ligase that promotes degradation of p53, thereby inhibiting its transcriptional activity [42]. Our results suggest that CENPN might regulate p53 expression through MDM2-mediated degradation. To further examine the relationship between CENPN and p53 transcriptional activity, we treated PAAD cells downregulated for CENPN with PFTα, a p53 inhibitor that inhibits its transcriptional activity by blocking its binding to DNA [43, 44]. The results showed that the effect of si-CENPN on promoting apoptosis of PAAD cells was weakened after treatment with PFTα, suggesting that CENPN-mediated apoptosis in PAAD cells may be related to p53 transcriptional activity. However, there are limitations to our study that should be recognized. The use of publicly available microarray data may be biased by small sample sizes and unconsidered confounding factors. Furthermore, although our cell line experiments revealed a role for CENPN, they did not fully replicate the complex in vivo tumor microenvironment.
In conclusion, our results suggest that CENPN may regulate the P53 signaling pathway in PAAD by affecting MDM2 expression and p53 transcriptional activity. However, further experimental and clinical validation is required to fully elucidate the potential mechanisms and therapeutic targets of PAAD. The multifaceted nature of PAAD calls for comprehensive studies to reveal its underlying complexity and develop effective therapeutic strategies.
The study employed bioinformatics to identify the key gene CENPN, associated with survival and prognosis, in patients with pancreatic ductal adenocarcinoma (PAAD). We observed a correlation between CENPN expression and TP53 gene mutation status, as well as an association between its high expression and increased drug sensitivity. In vitro experiments further demonstrated that overexpression or knockdown of CENPN affects cell proliferation, migration, and invasion capabilities. Moreover, the research revealed that CENPN regulates cell apoptosis in PAAD through the MDM2-mediated p53 signaling pathway. Collectively, these findings substantiate CENPN as a potential therapeutic target in PAAD treatment.